|
Accurate model predicting sustained response at week 4 of therapy with pegylated interferon with ribavirin in patients with chronic hepatitis C
|
|
|
Journal of Viral Hepatitis
Volume 13 Issue 10 Page 701 - October 2006
M. Martinot-Peignoux1, L. Comanor2, J. M. Minor3, M. P. Ripault1, B.-N. Pham4, N. Boyer1, C. Castelnau1, N. Giuily1, D. Hendricks5 and Patrick Marcellin1
"...., this study performed in routine patients showed that this multivariate model allows early prediction of SVR and NR at baseline as well as week 4 of therapy on naive as well as in previously treated patients.... we describe multivariate models that use the commonly collected data [i.e. sex, age, genotype, serum alanine aminotransferase (ALT) and HCV RNA levels, histologic necroinflammation and fibrosis scores and whether previously treated or naive] to predict response to peginterferon plus ribavirin therapy for patients in routine clinical practice..... The week 4 multivariate model included 26 terms, of which 3 were linear and 23 were combinatorial. The linear terms were the log of the viral load decline by week 4, log of the baseline viral load and sex, which ranked 1st, 3rd, and 20th, respectively, in relative importance to the week 4 model...."
Summary. Current models used to predict response to peginterferon plus ribavirin treatment, based on viral decline during the first 12 weeks of therapy, have focused on creating an early stopping rule to avoid unnecessary prolongation of therapy.
We developed a multivariate model that predicted sustained virological response and nonresponse at baseline and during the first 12 weeks of therapy using collected data from 186 unselected patients with chronic hepatitis C treated with peginterferon plus ribavirin. This model employed ordinal regression with similarity least squares technology to assign the probability of a given outcome.
Model variables include sex, age, prior treatment status, genotype, baseline serum alanine aminotransferase levels, histologic necroinflammation and fibrosis scores and serum hepatitis C virus RNA concentration at baseline and weeks, 4, 8, and 12.
A multivariate model demonstrated high performance values at all time points. At baseline, the model demonstrated a negative predictive value (NPV) and a positive predictive value (PPV) of 91% and 95%, respectively. At week 4, these values improved to 97% and 100%, respectively, with 95% sensitivity, 89% specificity and 93% accuracy. At week 4, the model was equally efficient for naive or previously treated patients. Internal validation demonstrated 90% PPV, 94% NPV, 95% sensitivity, 88% specificity and 92% accuracy.
A week 4 stopping rule for patients with chronic hepatitis C treated with peginterferon with ribavirin might be proposed by using the model developed in our study.
Introduction
Recently, there has been great emphasis on developing models for early prediction of therapeutic outcome for patients with chronic hepatitis C [1,2]. Although new formulations of peginterferon plus ribavirin treatment have increased the rate of sustained virological response (SVR) to 45-59% [2-4], moderate-to-severe side effects are common and the cost of treatment is still considerable. Ideally clinicians would like to assess the likelihood of SVR before treatment or as early as possible during its course, not only to discontinue treatment in those cases in which viral clearance likely is not going to occur but also to encourage compliance in patients likely to respond.
Currently, the most widely accepted models for the prediction of outcome to peginterferon plus ribavirin therapy were developed from data collected from two international registration trials and are based on early virological response. The definition of early virological response is a decline in serum hepatitis C virus (HCV) RNA greater than or equal to 2-log10 units or clearance of virus by a sensitive qualitative assay by week 12 of therapy. Univariate models based on this definition provide the basis for early stopping rules for peginterferon plus ribavirin therapy at week 12 [3,5]. These univariate models maximize the negative predictive value (NPV) in order to minimize loss of potential responders, as opposed to optimizing the positive predictive value (PPV) that would yield a higher rate of SVR among those continuing treatment but would exclude some patients who might ultimately respond. Recently, investigators have used multiple viral load measurements during the first month of therapy in an attempt to create a week 4 stopping rule [6]. However, as these univariate models are based only on virological response, they provide good NPV but poorer PPV, and they have low specificity and accuracy. In addition, they are unable to make baseline predictions.
We conducted a prospective community-based study to evaluate the relationship between viral kinetics and outcome to peginterferon plus ribavirin therapy. During the course of this study, we developed multivariate models to predict treatment outcome. Here, we describe multivariate models that use the commonly collected data [i.e. sex, age, genotype, serum alanine aminotransferase (ALT) and HCV RNA levels, histologic necroinflammation and fibrosis scores and whether previously treated or naive] to predict response to peginterferon plus ribavirin therapy for patients in routine clinical practice. We also evaluated the efficacy of these multivariate models by internal model validation.
Methods
Selection of patients
One hundred and eighty-six patients with chronic HCV infection (97 naive and 89 previously treated) treated with peginterferon plus ribavirin were prospectively included in this study. Of these, 73 (39%) received a reduced dosage of one or both drugs but finished the treatment course; they were included in the study, as dose reduction is common in clinical practice. All patients had elevated serum ALT levels, detectable anti-HCV antibodies and HCV RNA in serum at baseline and a liver biopsy performed within 12 months prior to the initiation of peginterferon plus ribavirin treatment. Patients were included for the purpose of model development only if they had completed a full course of therapy and had blood sampled in our centre for viral measurements at baseline and two or more time points during therapy (week 4, 8 and/or 12) at the end of treatment (EOT) and at the end of week 24 follow-up. The clinical characteristics of these patients are shown in Table 1. Of the 89 previously treated patients, 41 had received standard interferon monotherapy (3 MU, 3 times per week for 6 months), 3 peginterferon monotherapy (1.5 μg/kg/week) and 45 interferon and ribavirin (3 MU, 3 times per week for 6 months plus ribavirin 1000-1200 mg/day according to weight) as prior therapy. Sixty-three patients were previous nonresponders and 26 were previous relapsers to these prior therapies.
Treatment regimens and definition of response
Patients were treated with peginterferon α-2b (PEG-Intron; Schering Plough Research Institute, Kenilworth, NJ, USA) at a dosage of 1.5 μg/kg body weight per week and ribavirin (REBETOL; Schering Plough Research Institute) at a dosage of 800-1200 mg/day depending on body weight, according to the consensus guidelines [7,8]. Naive patients infected with genotypes 1, 4 or 5 and all previously treated patients were treated for 48 weeks; patients infected with genotypes 2 and 3 were treated for 24 weeks.
SVR was defined by undetectable serum HCV RNA after 24 weeks of follow-up. NR was defined by detectable serum HCV RNA at the EOT. RR was defined by undetectable serum HCV RNA at the EOT and by viral breakthrough during follow-up.
Virological, biochemical and histological evaluation
Serum HCV RNA was quantified by the VERSANT HCV RNA 3.0 (bDNA) Assay (Bayer Healthcare LLC, Tarrytown, NY, USA) with a quantification range of 615-7 690 000 IU/mL [9]. Serum samples below 615 IU/mL were evaluated with the VERSANT HCV RNA Qualitative Assay (HCV Qual TMA, Bayer Healthcare LLC) with a limit of detection of ≦9.6 IU/mL [10]. For modelling purposes, samples with viral measurements below 615 IU/mL but with detectable HCV RNA by HCV Qual TMA were assigned a value of 385 IU/mL (2000 copies/mL), whereas samples with undetectable HCV RNA by both assays were assigned a value of 19 IU/mL (100 copies/mL). HCV genotypes were identified using the VERSANT HCV LiPA (Bayer Healthcare LLC) [11]. Serum HCV RNA and ALT levels were determined at baseline, weeks 4, 8, 12, EOT and follow-up week 24. Liver biopsies were graded using the METAVIR scoring system [12].
Multivariate model development
A statistical data analysis network known as similarity least squares (SMILES or SLSTM) [13,14] was used to develop multivariate models for predicting likelihood of response to peginterferon plus ribavirin therapy. These multivariate models were designed to distinguish 'two outcomes'; SVR and nonsustained response at baseline and at weeks 4, 8 and 12. Whereas the baseline multivariate model used only variables known at baseline, multivariate models for weeks 4, 8 and 12 used all baseline variables plus viral load values at those time points. Continuous variables, such as viral load and serum ALT, were used directly. Nominal variables (i.e. those without inherent continuous properties), such as genotype and sex, were converted to continuous variables using ordinal logistic analysis to correlate nominal levels to probability of outcome (JMP 5.0.1.2; SAS Institute, Cary, NC, USA). Continuous variables were standardized to Z scores and entered in the models as linear terms. As is the standard statistical practice, all data were used for the univariate normalizations and nominal conversions.
Then SMILES was used to create combinatorial terms that incorporate combinations of linear terms into the model. A demonstration of the model is available on the web site http://www.slsguy.com. A subset of linear and combinatorial terms most important for prediction of response (essential terms) was selected using best subset selection techniques. In addition, a subset of patients necessary to produce a complete model (critical patients) was identified using methods similar to the principles of computational design of experiments (http://www.echip.com). With SMILES technology, the number of essential terms and critical patients required for each multivariate model varies according to the amount of information available to the model as well as the amount of predictive information required from the model. For example, baseline models require more essential terms and critical patients than models with additional information obtained during therapy (e.g. viral kinetic data).
Finally, ordinal logistic regression was used to determine the best linear combination of essential terms to predict the response of critical patients and optimized to allow for approximately five degrees of freedom from error. A scoring function created by SMILES was used to predict the response of all noncritical patients. The equation for the scoring function is:
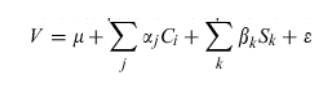
where V is the SMILES-derived metric used to predict the outcome for each patient where the collection {C} is a subset of clinical variables, Sk is the similarity between the patient profile and the profile of feature k where similarity can be any viable function of profile proximity and the Greek symbol (μ,α,β and ) parameters to be estimated or optimized from the training data composed of critical patients. V values of <-0.05 imply sustained response, values >0.1 imply NR and in-between values suggest that the patient is unpredictable, that is, he/she is on the defining boundary of interactions that divide sustained responders from nonresponders.
Assessment of model performance
The accuracy of the model was assessed by calculating the sensitivity, specificity, PPV and NPV at each time point. Sensitivity was calculated as the percentage of SVRs identified by the model [(number of correctly predicted SVRs/number of true SVRS) X 100]. Specificity was calculated as the percentage of NRs identified by the model [(number of correctly predicted NRs/number of true NRs) X 100]. PPV was calculated as the percentage of SVRs predicted by the model who are true SVRs [(number of correctly predicted SVRs/total number of predicted SVRS) X 100]. NPV was calculated as the percentage of NRs predicted by the model who are true NRs [(number of correctly predicted NRs ÷ total number of predicted NRs) X 100].
Model validation
The SLS system performs its own validation since it trains on 20% of the patients and subsequently predicts 80%. As an additional test of robustness internal model validation was performed by creating a multivariate model from a training set comprised of a random selection of 80% of the patients, and then testing the performance of this model in predicting the response of the remaining 20% of patients. Week 4 was chosen as the time point for internal model validation to assess the feasibility of creating a week 4 stopping rule.
Results
Patient response to treatment
Baseline characteristics and response to treatment for the 186 patients are shown in Table 1. The overall sustained response rate was 55%. The 48% sustained response rate among naive patients was not statistically different from the 62% rate observed in previously treated patients. As expected, previously treated patients who did not respond to prior treatment had a lower rate of SVR than those who relapsed after prior treatment. Of the 63 patients who did not respond to prior treatment, 39 did not respond and 24 achieved SVR when subsequently treated with peginterferon plus ribavirin. Of the 26 patients who relapsed after prior treatment, 5 relapsed again, 2 did not respond and 19 achieved SVR after treatment with peginterferon plus ribavirin. In addition, a greater SVR rate to peginterferon plus ribavirin therapy was observed among patients who previously had received standard interferon monotherapy (54%) as compared with those previously treated with standard interferon plus ribavirin (40%). All three patients who received previous treatment with peginterferon monotherapy achieved SVR upon subsequent treatment with peginterferon plus ribavirin.
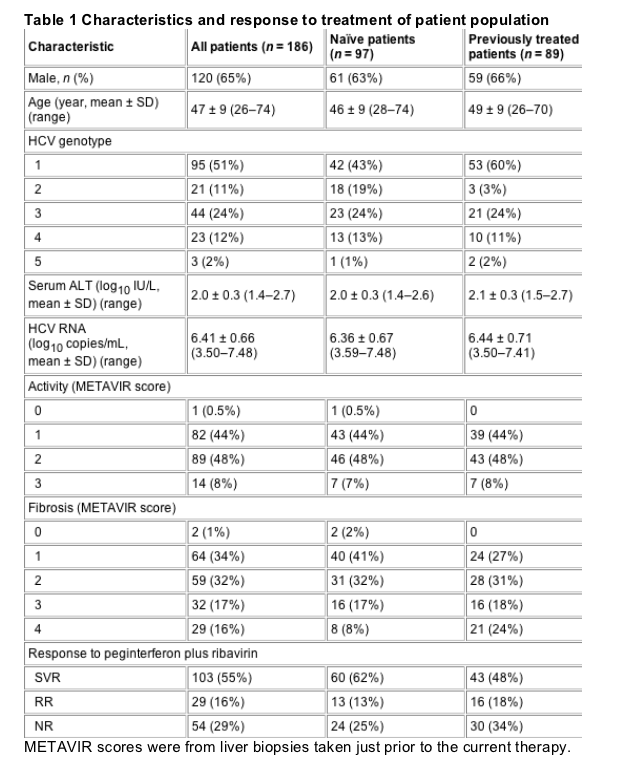
Performance of the models
Model performances are shown in Table 2. Even with the limited information available at baseline, the model demonstrated, 94% PPV, 91% NPV, 95% sensitivity, 89% specificity and 93% accuracy. At all time points during therapy, multivariate models showed consistently high sensitivity (98-100%), specificity (100%) and accuracy (99-100%). The performances of the multivariate model for naive patients vs previously treated patients at baseline and week 4, the only time points at which the performance of the multivariate models was less than 100%, are shown in Table 3.
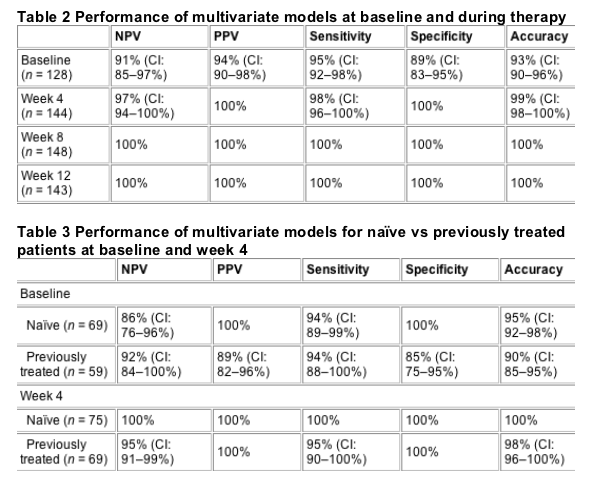
Relative contribution of clinical variables
To understand the relative contribution of the clinical variables in the multivariate models, logistic regression analysis was performed to rank the impact of individual clinical variables that are explicit in the model (i.e. linear terms) and the impact of combinatorial effects among all the clinical variables (i.e. combinatorial terms). The baseline multivariate model included 48 terms, of which 3 were linear and 45 were combinatorial. The linear terms were HCV genotype, log of the baseline viral load, and fibrosis score, which ranked 5th, 16th and 28th, respectively, in relative importance to the baseline model. The week 4 multivariate model included 26 terms, of which 3 were linear and 23 were combinatorial. The linear terms were the log of the viral load decline by week 4, log of the baseline viral load and sex, which ranked 1st, 3rd, and 20th, respectively, in relative importance to the week 4 model.
Performance of validation model
The week 4 model validation, performed on 36 patients, demonstrated a NPV of 94% (CI: 88-100%), a PPV of 90% (CI: 81-99%) with 95% (CI: 88-100%) sensitivity, 88% (CI: 77-99%) specificity and 92% (CI: 86-98%) accuracy. The overall high performances of the model (88-95%) upon validation demonstrate its robustness. A retrospective analysis of the 36 patients used to test the multivariate model revealed that the model correctly predicted the response of 3 of 7 critical patients and all 29 noncritical patients. In addition, the model correctly predicted the response of 20 of 21 naive patients and 13 of 15 previously treated patients. Of 36 patients used to test the multivariate model, 12 had received reduced dosages of peginterferon and/or ribavirin and the model correctly predicted 11 of these 12 patients.
Discussion
Previously developed univariate models for prediction of response to peginterferon plus ribavirin were based on retrospective studies using data from two international registration trials of that therapy [3,4]. These registration trials admitted only naive patients who were capable of receiving an optimal treatment regimen. By contrast, our multivariate model was based on data from a prospective community-based study that included nearly equal numbers of naive and previously treated patients. The univariate models developed using data from the registration trials were optimized for high NPV and thus are well suited for identifying patients not likely to respond to therapy [3,4]. However, these models have poor specificity and therefore cannot be used with certainty to predict SVR.
Our multivariate model based on SMILES technology was optimized for accuracy instead of NPV. At baseline, our multivariate model had excellent values for all five performance attributes (89-95%), allowing prediction of response using data available prior to starting therapy. At week 4 of therapy, our model showed performance values ranging from 97 to 100%. The robustness of this model is indicated by its high performance values upon internal validation ranging from 88 to 95%. Unlike univariate models, the predictive ability of our multivariate models can be further improved by the addition of more patient data.
The SMILES process allows us to model effectively a heterogenous population using a data set based on 186 patients, whereas a conventional logistic regression modelling approach for this population would require more data. Our multivariate models use patient profiles instead of individually weighted variables to predict outcome, allowing relatively few critical patients to predict the response of the remaining patients. The efficiency of the week 4 multivariate model is demonstrated by its ability to optimise all 26 model parameters based on a minimum of 30 patient profiles selected by design of experiment techniques. Incorporation of these designs of experiment concepts along with the ability to model combinatorial effects make this efficiency possible. The limitation of building our multivariate model with only 186 patients lies in not knowing if all the important interactions have been accurately captured from the available patient data.
Another advantage of this kind of multivariate model is its ability to deal in part with missing data. Incorrect data is the worst kind of missing data and the SMILES technology is useful in identifying data errors during data preprocessing. In addition, in this type of modelling, imputation can be used to calculate insertions for specific values if the percentage of actual missing data is small. Although these insertions do not create the missing information, they do enable the effective use of the known data in a profile. The impact of missing data depends on the importance to the model of missing variables. Absence of a critical variable such as viral load will make a large difference, whereas absence of a minor one will have less impact. Nevertheless, even a minor variable can assume importance in combination with another variable.
The variables used in our study have been shown to be independent predictors of response in previous studies, [1,5,6,15-20]. They reflect both host and viral factors such as genotype, age, sex, serum ALT, fibrosis score and viral decline. Several of these variables proved to be important as linear terms in our model. Because our multivariate model also included prior treatment status as a variable, it can be applied to both naive and previously treated patients and, in fact, performed well in predicting the response of both patient groups.
Unlike univariate models, the predictive capability of our multivariate model relies heavily on combinatorial terms. The potential utility of combinatorial terms in prognostic models has been suggested in an earlier study of patients treated with interferon-based therapies that found a correlation between the number of predictive factors and the response rate [21]. The more positive predictive factors a patient had, the more likely he/she was to achieve SVR. Conversely, response was synergistically impaired when two or more negative predictive factors were present. While these results indicate that interactions between two or more clinical variables are important in predicting response, it is not practical for the physician to weigh and evaluate the large number of possible interactions between clinical variables in predicting response for a given patient. The use of patient profiles instead of individually weighted variables makes it possible for SMILES to correctly predict difficult patients. For example, four of our correctly predicted sustained responders were nonresponders to previous treatment, infected with genotype 1, had above average baseline viral load and less than a 2 log decline in viral load by week 4. However, the model correctly captured the interactions of other variables such as fibrosis and inflammatory scores and ALT, which enabled these patients to respond.
Finally, the design of the multivariate model gives it inherent flexibility that allows incorporation of new information, such as genomic data, treatment compliance, physician monitored dose modifications, body mass index, etc. Flexibility is an important advantage for prognostic models, as evaluation, therapy and monitoring of chronic hepatitis C patients is continuously evolving. Although the presented models are specific to the data they were trained on, with minor modifications they may be applied to slightly different data. For example, the model could be tested on a new set of patients taking a different regimen of interferon and an extrapolation could be developed.
In conclusion, this study performed in routine patients showed that this multivariate model allows early prediction of SVR and NR at baseline as well as week 4 of therapy on naive as well as in previously treated patients. Prediction of SVR may provide patients with an early incentive for treatment compliance, a critically important factor during the first week of therapy. In this way, use of the model could lead to increased sustained response. Prediction of NR may allow discontinuation of therapy with confidence or may suggest a modification of the current regimen.
References
1 Berg T, Sarrazin C, Herrmann E et al. Prediction of treatment outcome in patients with chronic hepatitis C: significance of baseline parameters and viral dynamics during therapy. Hepatology 2003; 37: 600-609.
2 Davis GL, Wong JB, McHutchison JG, Manns MP, Harvey J, Albrecht J. Early virologic response to treatment with peginterferon alfa-2b plus ribavirin in patients with chronic hepatitis C. Hepatology 2003; 38: 645-652.
3 Manns MP, McHutchison JG, Gordon SC et al. Peginterferon alfa-2b plus ribavirin compared with interferon alfa-2b plus ribavirin for initial treatment of chronic hepatitis C: a randomised trial. Lancet 2001; 358: 958-965.
4 Fried MW, Shiffman ML, Reddy KR et al. Peginterferon alfa-2a plus ribavirin for chronic hepatitis C virus infection. N Engl J Med 2002; 347: 975-982.
5 Davis GL. Monitoring of viral levels during therapy of hepatitis C. Hepatology 2002; 36: S145-S151.
6 Neumann A, Schalm S, von Wagner M et al. Early viral kinetics prediction of sustained virological response after 1 or 4 weeks of PEG-interferon-alfa-2a and ribavirin therapy (DITTO HCV-project). Hepatology 2003; 38 (Suppl.): 248A (Abstract).
7 National Institutes of Health. Management of hepatitis C: 2002 NIH Consens State Sci Statements 2002; 19(3): 1-46. Accessed at: http://consensus.nih.gov/cons/116/116cdc_intro.htm.
8 Dhumeaux D, Marcellin P, Lerebours E. Treatment of hepatitis C. The 2002 French consensus. Gut 2003; 52: 1784-1787.
9 Elbeik T, Surtihadi J, Destree M et al. Multicenter evaluation of the performance characteristics of the Bayer VERSANT HCV RNA 3.0 assay (bDNA). J Clin Microbiol 2004; 42: 563-569.
10 Comanor L, Anderson F, Ghany M et al. Transcription-mediated amplification is more sensitive than conventional PCR-based assays for detecting residual serum HCV RNA at end of treatment. Am J Gastroenterol 2001; 96: 2968-2972.
11 Stuyver L, Rossau R, Wyseur A et al. Typing of hepatitis C virus isolates and characterization of new subtypes using a line probe assay. J Gen Virol 1993; 74: 1093-1102.
12 Bedossa P, Poynard T. An algorithm for the grading of activity in chronic hepatitis C. The METAVIR Cooperative Study Group. Hepatology 1996; 24: 289-293.
13 Comanor L, Minor LM. Methods and apparatus for predicting therapeutic outcomes. US5860917A1, Chiron Corporation, 1999.
14 Minor JM, Namini H. Analysis of clinical data using neural nets. J Biopharm Stat 1996; 6: 83-104.
15 Zeuzem S. Heterogeneous virologic response rates to interferon-based therapy in patients with chronic hepatitis C: Who responds less well? Ann Intern Med 2004; 140: 370-381.
16 Martinot-Peignoux M, Marcellin P, Pouteau M et al. Pretreatment serum hepatitis C virus RNA levels and hepatitis C virus genotype are the main and independent prognostic factors of sustained response to interferon alfa therapy in chronic hepatitis C. Hepatology 1995; 22: 1050-1056.
17 Martinot-Peignoux M, Boyer N, Pouteau M et al. Predictors of sustained response to alpha interferon therapy in chronic hepatitis C. J Hepatol 1998; 29: 214-223.
18 Lee SS, Heathcote EJ, Reddy KR et al. Prognostic factors and early predictability of sustained viral response with peginterferon alfa-2a (40 KD). J Hepatol 2002; 37: 500-506.
19 Marcellin P, Pouteau M, Martinot-Peignoux M et al. Lack of benefit of escalating dosage of interferon alfa in patients with chronic hepatitis C. Gastroenterology 1995; 109: 156-165.
20 McHutchison JG, Gordon SC, Schiff ER et al. Interferon alfa-2b alone or in combination with ribavirin as initial treatment for chronic hepatitis C. Hepatitis Interventional Therapy Group. N Engl J Med 1998; 339: 1485-1492.
21 Poynard T, Marcellin P, Lee SS et al. Randomised trial of interferon alpha-2b plus ribavirin for 48 weeks or for 24 weeks versus interferon alpha-2b plus placebo for 48 weeks for treatment of chronic infection with hepatitis C virus. International Hepatitis Interventional Therapy Group (IHIT). Lancet 1998; 352: 1426-1432.
|
|
|
|
|
|
|