 |
 |
 |
|
HBV DNA & Genotype Predict HCC
|
|
|
Reported by Jules Levin
DDW, May 19-24, 2007, Washington DC
"An HCC Risk Function Nomogram for Patients With
Chronic Hepatitis B: The R.E.V.E.A.L.-HBV Study"
"...The combination of HBV DNA ≥105 copies/mL (100,000) and genotype C had the highest HCC risk...."
C-J Chen1,2, H-I Yang1,2, UH Iloeje3, J Su3, C-L Jen1,2, S-L You1, M Sherman4, Y-F Liaw5, P-J Chen2
1Genomics Research Center, Academia Sinica, Taipei, Taiwan; 2National Taiwan University, Taipei, Taiwan; 3Bristol-Myers Squibb Pharmaceutical Research Institute, Wallingford, CT, USA; 4University of Toronto, Toronto, ON, Canada; 5Chang Gung Memorial Hospital and University, Taipei, Taiwan
Abstract
Background and Aims: Counselling chronic hepatitis B (CHB) patients about their individual risk of progressing to liver complications is a clinical challenge. Our objective was to develop a simple risk function nomogram using noninvasive clinical information in CHB-infected subjects.
Methods: The following risk factors were used: gender, age, cigarette smoking, alcohol consumption, family history of hepatocellular carcinoma (HCC), ALT, HBeAg, HBV DNA, HBV genotype, basal core promoter (BCP) and pre-core mutations. Cox proportional hazards models were used to train models. Regression coefficients derived from the Cox models were converted into risk scores and the predicted risks of HCC over 5 and 10 years calculated. The risk scores and the predicted 5- and 10-year HCC risks were translated into nomograms. The predictive accuracy was evaluated using Receiver Operator Characteristic (ROC) curve and area under the ROC curve (AUROC), and the calibration chart.
Results: N = 3644; mean follow-up time was 11 years.
The best model had an AUROC of 0.8601 (5-year HCC prediction) and 0.8649 (10-year HCC prediction).
Males were at higher risk of HCC than females.
For all HBV DNA levels, genotype C patients had a higher risk of HCC than genotype B patients.
The nomogram derived from the model had good calibration and the predicted
risk approximated the actual risk.
Conclusions: The predictive model has good characteristics as shown by the AUROC. The combination of HBV DNA ≥105 copies/mL (100,000) and genotype C had the highest HCC risk. The derived nomogram, once validated, should simplify the communication of individual HCC risk using noninvasive variables.
Introduction
Several important predictors of HCC risk in patients with chronic hepatitis B have
been identified
Well-designed clinical trials have shown that various drug therapies that reduce these factors decrease the risk of some HCC events
Predicting patients at risk of progressing to HCC may help in patient counselling, risk stratification and identifying those most likely to benefit from intervention
Data from our long-term, population-based R.E.V.E.A.L.-HBV studies might be used to estimate the risk of individuals chronically infected with HBV for developing HCC and the likelihood of benefit from treatments over a specific time frame
The objective of this analysis was to develop a simple risk function nomogram using noninvasive clinical information in CHB-infected subjects
Methods
- Source of data: 3,644 anti-HCV-negative participants with CHB (aged 30-65 years) in the R.E.V.E.A.L.-HBV cohort (Figure 1)
Figure 1: Flow of Study Subjects
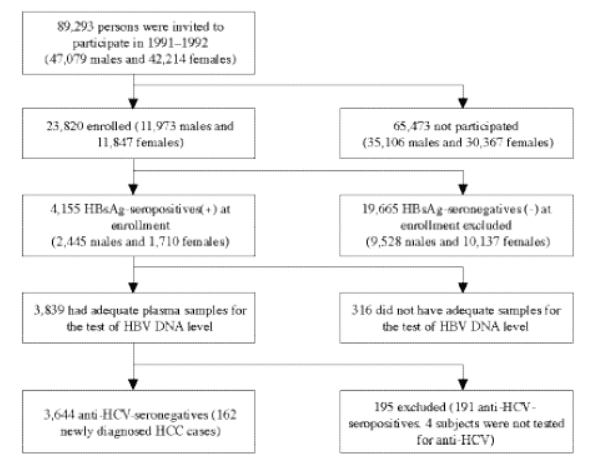
Baseline variables used:
- From questionnaire: gender, age, alcohol consumption, family history of HCC
- Laboratory testing:
- HBeAg by radioimmunoassay (Abbott Laboratories, North Chicago, IL)
- ALT by serum chemistry autoanalyzer (Model 736, Hitachi, Tokyo, Japan) using commercial reagents (bioMérieux, Marcy L'Etoile, France)
- HBV DNA by real-time PCR analysis
- HBV genotype by melting curve analysis
- HBV pre-core 1896 and basal core promotor 1762/1764 mutants by direct sequencing analysis
Multivariable Cox proportional hazards modelling was used to train the model. The regression coefficients derived from the Cox models were then converted into integer risk scores (a rounding of the exact _-coefficient obtained from the model)
Predicted 5- and 10-year HCC risk for the scoring system using the following formula and then incorporated the predicted risk and scoring system into nomograms1
-- P redicted 5/10-year HCC risk = 1-(1-P0)e x p ( R S - R S 0 )
-- P0: Predicted probability of HCC within 5/10 years for persons with the reference-level risk score RS0
-- RS: Risk score of which HCC risk to be predicted
Predictive accuracy was assessed by evaluating the discrimination and calibration abilities of models
Discrimination ability was assessed by using ROC curve and area under ROC curve (AUROC)
Calibration ability was evaluated by using calibration chart
RESULTS
Considering the feasibility in various clinical settings, 8 models were developed (Table 1). Results of Models 1, 5, and 8 are shown.
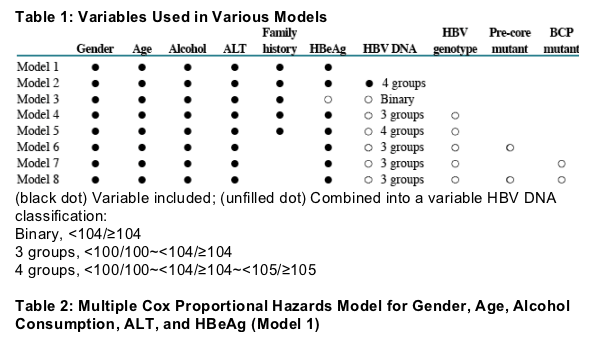
Table 3: Multiple Cox Proportional Hazards Model for Gender, Age, Alcohol
Consumption, ALT, HBeAg, and the Combinations of HBV DNA and Genotype (Model 5)
HBV DNA / genotype (referent: <100 / not tested):
100 to <10,000 / B: Hazard Ratio 2.2 (0.9-5.3) p 0.0700
100 to <10,000 / C: HR 3.8 (1.6-9.3) p 0.0028
10,000 to <100,000 / B: HR 3.0 (1.3-6.9) p0.0088
10,000 to 100,000 / C: HR 8.6 (3.6-20.9) p <0.0001
>100,000 / B: HR 7.1 p <0.0001
>100,000 / C: HR 10.9 (5.0-24.1) p <0.0001
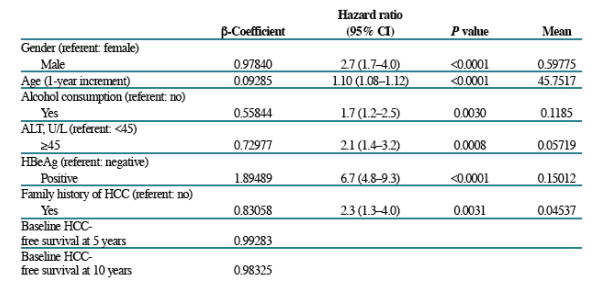
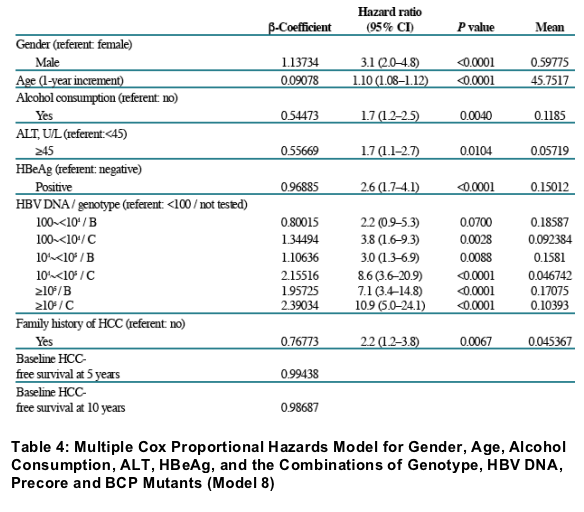
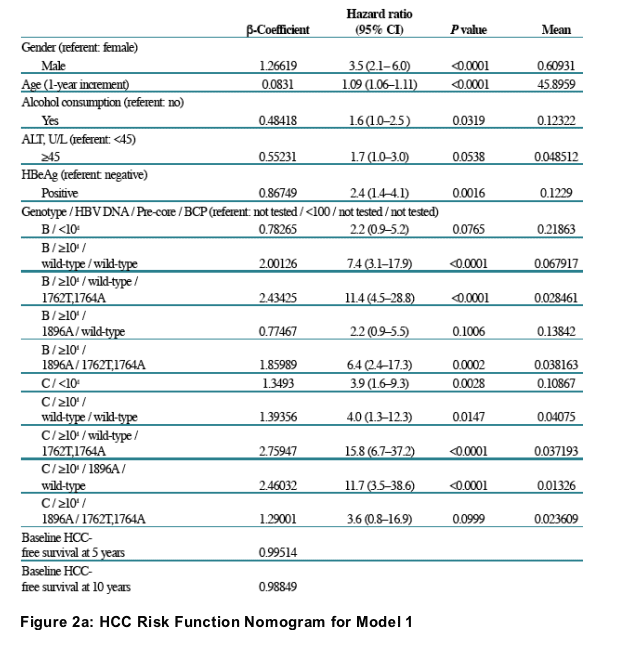
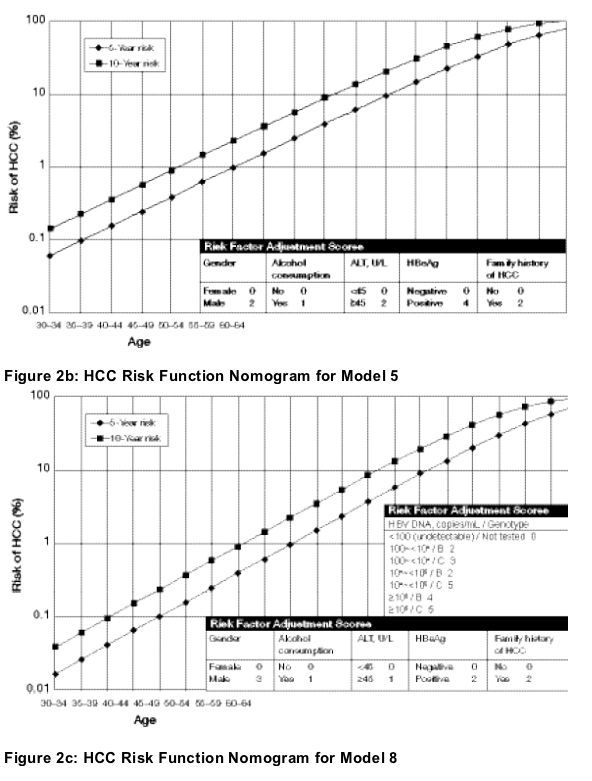
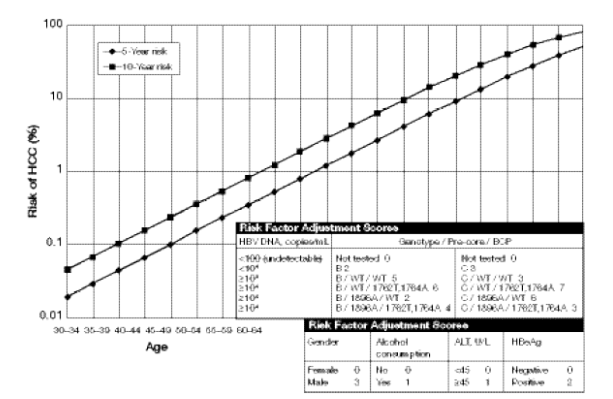
Methods for using the nomogram:
- Locate the subject's age group from the horizontal scale
- Trace upward to the corresponding rhombus or square on the 5- or 10-year risk line
- Taking other risk factors into consideration:
Check the risk factor adjustment score in the box at right bottom side according to each risk factor present in the subject
Move to the right up the risk line the appropriate number of square or rhombus (each square or rhombus equals 1 point)
Repeat this step in series for each risk factor. Once all risk factors have been considered, find the corresponding value on vertical scale to determine the subject's estimated risk
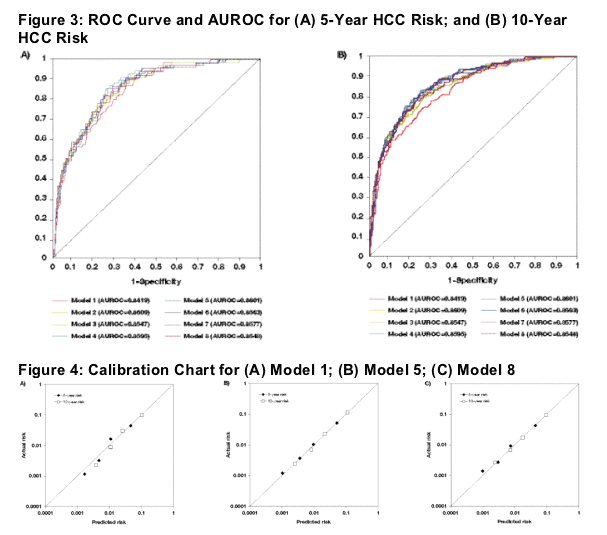
CONCLUSIONS
The nomograms offer a means of making a fast, reasonable and visually explicit risk estimation of HCC
The models in this study could also be used to generate a patient risk calculator, using other commonly available software and tools
The models had good discrimination and calibration abilities. Once passing external validation, these models should simplify the communication of individual HCC risk using noninvasive variables
References
1. McCormack JP, Levine M, Rangno RE. Primary prevention of heart disease and stroke: a simplified approach to estimating risk of events and making drug treatment decisions. CMAJ. 1997;157(4):422-428.
Financial Disclosures
Chein-Jen Chen: (BMS) Research Grant; Hwai-I Yang: None; Uchenna H. Iloeje: (BMS) Employee; Jun Su: (BMS) Employee;
Chin-Lan Jen: None; San-Lin You: None; Morris Sherman: (BMS) Speaker, Advisory Board Member; Y-F Liaw: (Roche, BMS, GSK, Idenix) Research Support, Advisory Board Member and Speaker; P-J Chen: None
|
|
|
 |
 |
|
|