 |
 |
 |
|
Genotypic tipranavir scores as predictors of response
|
|
|
Reported by Jules Levin
11th European AIDS Conference (EACS),
Madrid, Spain
24-27 October 2007
J Schapiro1, J Scherer2, R Vinisko2, VM Kohlbrenner2, JD Baxter3,
CAB Boucher4, RD MacArthur5, DB Hall2
1 National Hemophilia Center, Israel
2 Boehringer Ingelheim Pharmaceuticals, Ridgefield, CT, United States
3 Cooper Hospital/UMDNJ-Robert Wood Johnson Medical School, New Jersey, United States
4 Department of Virology, University Medical Center Utrecht, the Netherlands
5 Wayne State University, Detroit, MI, USA
Introduction
Many genotypic scores have recently been developed to predict phenotype and/or response to treatment with tipranavir. An evaluation of how well these scores predict virologic response to tipranavir in the RESIST trials should provide some direction regarding their applicability.
Author Discussion
- Scores developed on large databases involving weighting based on sophisticated methodology do best at correlating with and predicting virologic response.
- As data becomes available, scores should be based on adequate samples within the correct population of patients. Otherwise, there is an increased likelihood of misclassifying a patient as resistant when susceptible or vice versa.
- There is a lack of consistency across scores in defining clinical cut-offs. It is clear that the cut-offs for Rega, for example, overestimate the percentage of patients resistant to tipranavir, resulting in over 50% of patients being declared resistant. These cut-offs limit the clinical applicability of the score for a population with experience similar to the RESIST patients.
- Further investigation of performance in more experienced patients, patients receiving potent background regimens, and patients with non-B subtypes of HIV-1 will be important for understanding response.
- Comparison of scores for multiple competing protease inhibitors will help differentiate between scores with respect to ability to identify the best drug to use.
- The predictive value of the Virtual Phenotype and the weighted score developed by Parkin imply that phenotype can be used as a surrogate for response when developing a score. Whether to test genotype, phenotype, or both when choosing a treatment regimen for an experienced patient cannot be addressed with available data.
Author Conclusions
- The tipranavir weighted score, Virtual Phenotype and the weighted score developed by Parkin appeared to be the best at predicting virologic response with TW and VP having the strongest correlations with viral load change from baseline.
- Simple scores were developed by MacArthur and Marcelin/Calvez to predict virologic response. Both include mutations given relatively high weights in the weighted scores and maintain some predictive value for this study population. Both of these scores were developed on a small population of patients. The same methodology could be applied a larger population to provide further insight into genotypic relationships with virologic response.
- The scores developed by Stanford, the Rega Institute and the tipranavir unweighted score performed similarly.
- Stanford weights given to several mutations, including V32, 54L and 90M, are discrepant with most other scores.
- The existing cut-offs for the Rega Institute overpredict resistance to tipranavir, with over 50% of patients being predicted to be fully resistant.
- Correlations of score versus response were stronger and roughly the same relationships held when examining patients with less than a log of predicted OBR support.
Methods
In the RESIST trials 745 patients were treated with TPV/r and had evaluable results for treatment response and baseline genotype. The TPV mutation scores compared in this study are provided below in Table 1.
Table 1: Summary of various tipranavir mutation scores compared with objective of the score and cut-offs used for clinical interpretations of resistance.
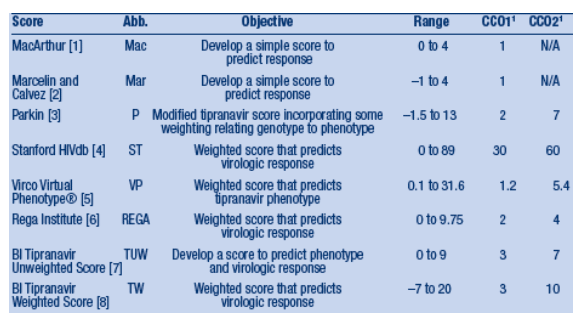
1CCO = Clinical Cut-Off: if score ≦CCO1, then the virus is predicted to be fully susceptible to tipranavir; if CCO1 < score ≦ CCO2 then virus is predicted to be partially susceptible to tipranavir; if score > CCO2 then virus is predicted to be resistant to tipranavir. Only one cut-off was suggested by MacArthur and Marcelin/Calvez due to the small range of their respective scores. So, for these scores the patient is either predicted to be susceptible or resistant depending on the score being ≦ or > than CCO1. Note also that the Stanford score provides 5 categories of resistance in intervals of 15, but these were collapsed to 3 for comparison purposes.
To compare the prediction accuracies of each score, week 8 (≥1 log drop in HIV RNA), 24 and 48 (HIV RNA<50 copies/mL) virologic response associations with each score were compared using logistic regression (assessed by areas under the receiver operator curves (AUROC)), adjusted for background drug activity (see below for a brief description). Spearman correlations of each score (R) with weeks 8, 24 and 48 viral load decline were also compared. These comparisons were made for all TPV/r-treated patients in RESIST and also for those with an
OBR predicted to provide less than one log of activity which would exclude, among others, those patients including new enfuvirtide in their regimen.
Comparisons were also made using the interpretation algorithms shown above in Table 1. An attempt was made to determine optimal cuts using the same methodology used to arrive at the clinical cut-offs for the BI tipranavir weighted score [8]. Comparisons of the predictive accuracy based on the existing cut-offs to our proposed cut-offs are shown as well as comparisons amongst the scores using the new cut-offs.
Background activity scores
The development of the weighted score and (model-based) comparisons to other scores were all adjusted for the activity of the background regimen. The estimated activities for each patient were based on a novel approach developed by Hall et al [9] that estimates the contribution of each drug in the Optimized Background Regimen (OBR) using a linear model-based approach accounting for the baseline resistance (using Virtual PhenotypeŽ) and historical use of each drug. Often, the activity of the drug in the background regimen, especially with the NRTIs, is largely dependent on the previous pattern of use rather than the baseline resistance. These adjustments prove to be much better at predicting response than a simple count of the number of active drugs in the OBR.
Results
Comparisons of score weights and score correlations
Table 2 shows all amino acid substitutions that are given a weight in each of the 8 scores compared. Mutations that appear in at least 5 of the 8 scores are 33F, 36I, 43T, 46L, 47V, 54A/M/V, 58E, 82L/T and 84V. Some notable differences across scores are:
- Mutations at V32 receiving large weights in the Stanford score and little inclusion in other scores.
- 54L receives a weight of 5 by Stanford but a negative weight by Parkin (-1) and large negative weight by the TW score (-7)
- 74P and 83D, fairly rare mutations, receive much larger (relative) weights by TW than the other scores
- 84V is strongly associated with changes in phenotype but less of a relationship is seen with virologic response, thus receives discrepant relative weights with larger weights given by P, ST and REGA compared to TW.
- 90M is given a relatively large weight by ST but only little consideration by the other scores.
Table 3 shows the pairwise correlations of the 8 scores. The strongest correlations were between REGA with P (0.84) and ST (0.83). The TW correlated most strongly with P (0.76) with correlations with both simple scores similar (0.39 for both) and also similar correlations with the other scores (ranging from 0.60 to 0.67). The Parkin score objective was similar to TW in that it weighted some existing TPV score mutations and added a few mutations that increased response so the fact that it correlated better with TW than TUW is reasonable.
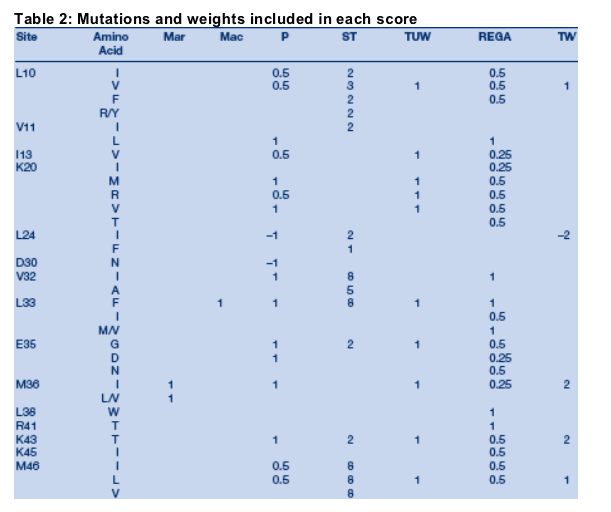
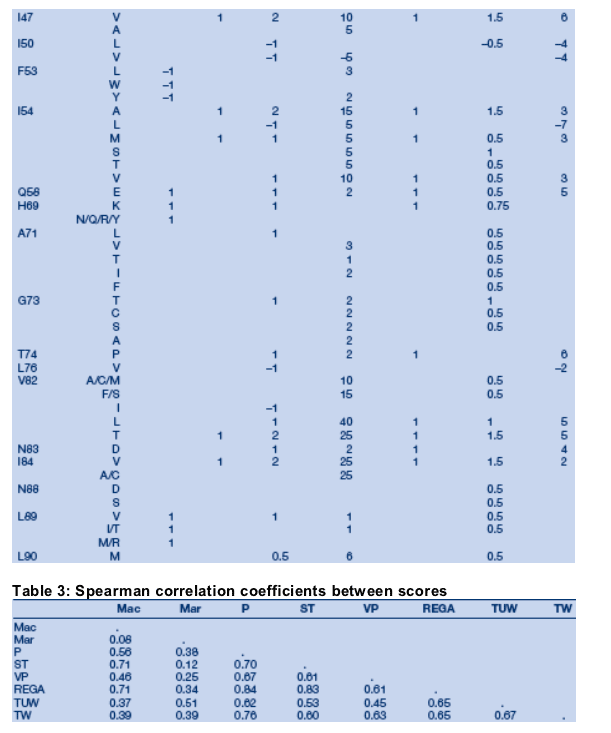
Comparison of prediction accuracies and correlations with virologic response
Tables 4 and 5 show the comparison summaries for all patients (Table 4) and patients without at least a log of antiviral activity from their OBR (Table 5). Figures 1 and 2 show the Receiver Operator Curves for the 5 weighted scores. The results are consistent and can be summarized as follows:
- Virtual Phenotype, the BI tipranavir weighted score and Parkin's weighted score perform the best.
- The unweighted tipranavir score and weighted scores by Stanford and the Rega Institute do not perform as well but do better than the simple scores developed by MacArthur and Marcelin/Calvez.
- The predictions and correlations for all scores, but especially TW and VP, are stronger for the patients with less OBR support.
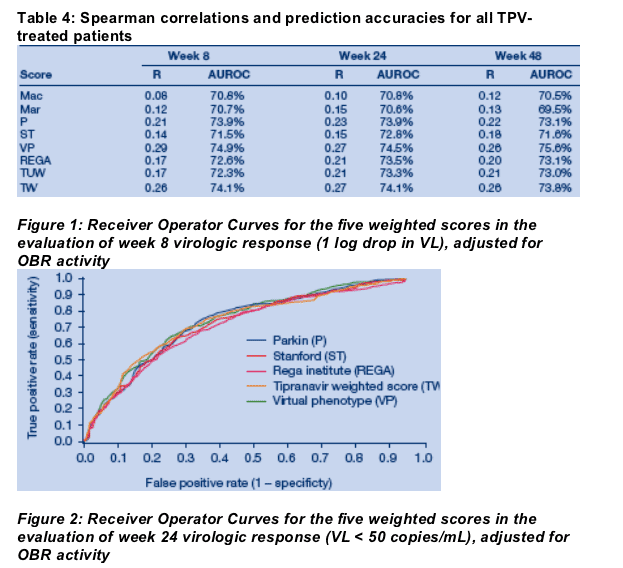
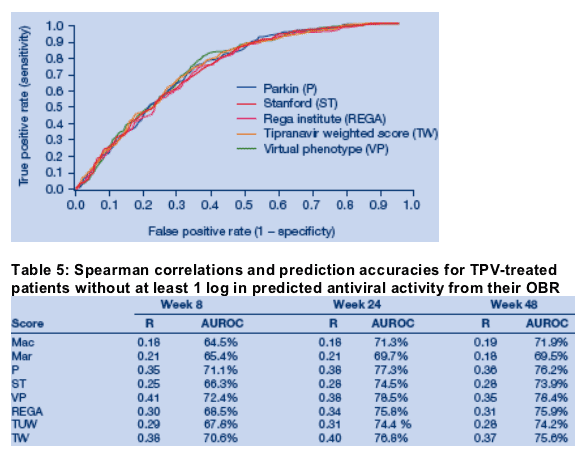
Response rates by existing cut-offs and comparison with proposed cut-offs
The response rates by clinical interpretation of each score are presented for week 24 response in Figure 3. The results of the search for optimal cut-offs for each score are presented in Table 6. A few key points about these results:
- The Rega Institute predicts 52% of patients to be fully resistant to tipranavir and only 10% to be susceptible. It is clear that their cut-offs need to be revised.
- The lack of score range for MacArthur and Marcelin/Calvez, due to their simplicity, also results in an overprediction of resistance, especially for MacArthur.
- The percentage of responders for those patients predicted to be susceptible is highest for the BI tipranavir weighted score while maintaining a low percentage of responders in the group of patients predicted to be resistant (of 95 patients predicted by TW to be resistant to tipranavir, only 6 responded).
- In general, the existing cut-offs were similar to the new cut-offs proposed in Table 5. Rega's cut-offs are the exception, though, with proposed cut-offs shifting considerably from the existing cut-offs.
- After applying the same methodology to each score to arrive at optimal cut-offs, the BI tipranavir weighted score clinical interpretation outperformed the other scores in terms of accurately predicting week 24 virologic response.
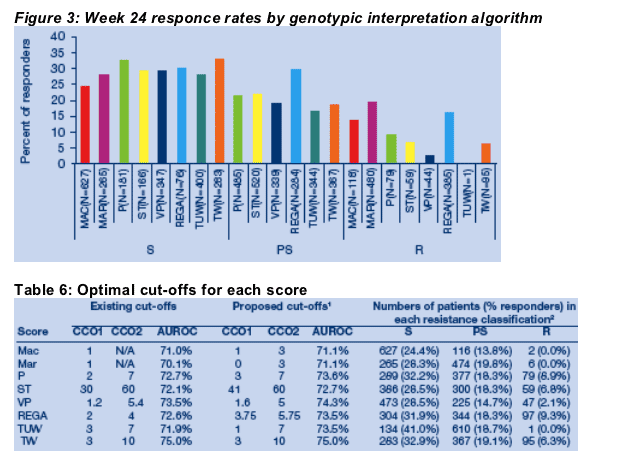
1 Proposed cuts are derived by fitting a logistic regression model of week 24 virologic response (VL <50) versus all possible cut-offs for each mutation score, adjusted for predicted OBR antiviral activity. The cut-offs that result in the largest prediction accuracy (measured by AUROC), with the constraint of having a false negative rate <10% (i.e. % responders with score > CCO2 is less than 10%).
2 Using the proposed cut-offs presented are the number of patients in each classification. The percentage of patients that had VL <50 within each classification are provided in parentheses (e.g. there were 304 patients predicted to be susceptible by the REGA proposed cut-offs and 31.9% of these patients had VL <50 at week 24).
References
1. MacArthur RD, et al. Drug Therapy in HIV Infection 2006, Glasgow (Poster #P205)
2. Marcelin AG, et al. 14th Conference on Retroviruses and Opportunistic Infections / CROI, 2007, Los Angeles, CA (Poster #612)
3. Parkin N, et al. 13th Conference on Retroviruses and Opportunistic Infections / CROI, February 5-8 2006, Denver, CO (Poster #637)
4. Stanford University HIV Drug Resistance Database
5. Vermeiren H, et al. Journal of Virologic Methods 2007; 145: 47-55
6. Algorithm for the use of genotypic HIV-1 resistance data, Rega v7.0 , 6 March 2007
7. Baxter JD, et al. Journal of Virology 2006; 80:10794-801
8. Scherer J, et al. 11th European AIDS Conference / EACS, October 24 - 27 2007, Madrid, Spain (Poster # P3.4/07)
9. Hall DB, et al. 11th European AIDS Conference / EACS, October 24 - 27 2007, Madrid, Spain (Poster # P4.3/71)
|
|
|
 |
 |
|
|