 |
 |
 |
|
Improving the prediction of virologic response to tipranavir:
the development of a tipranavir weighted score
|
|
|
Reported by Jules Levin
11th European AIDS Conference (EACS),
Madrid, Spain
24-27 October 2007
J Scherer1, CA Boucher2, JD Baxter3, JM Schapiro4, VM Kohlbrenner1, DB Hall1
1 Boehringer Ingelheim Pharmaceuticals, Ridgefield, CT, USA;
2 Department of Virology, University Medical Center Utrecht, the Netherlands;
3 Cooper Hospital/UMDNJ-Robert Wood Johnson Medical School, New Jersey, USA;
4 National Hemophilia Center, Israel
Introduction
The methodologies used to develop mutation scores to predict susceptibility to individual antiretroviral agents (ARVs) is in constant revision and thus it is not surprising, that when new methodologies are also applied to the original tipranavir score [1] the impact of the individual mutations identified are not the same, and appropriate adjustments for the contribution of each mutation towards virologic response are necessary. Also, it has been observed that several
mutations result in increased response or reduced likelihood of virologic failure and these mutations also need to be considered in the development of a mutation score to determine as accurately as possible the level of activity of a specific ARV. The purpose of this work was to develop weights for each of these mutations to arrive at a weighted TPV mutation score that will provide guidance to treating physicians on the impact of individual protease mutations on TPV activity.
Author Conclusions
- A tipranavir weighted score to predict virologic response was developed using the data from the RESIST trials.
- A few existing TPV score mutations, most of which are uncommon in patients who have not used TPV, have the greatest weights (47V, 54A/M/V, 58E, 74P, 82L/T, 83D) while the others were considered minor or not important in terms of accurately predicting virologic response.
- A score based entirely on mutations that are associated with reduced susceptibility will not predict response well for PI experienced patients. Mutations associated with increased susceptibility to tipranavir (24I, 50L/V, 54L, 76V) remained in the final score with large negative weights.
- Tested on an independent dataset against other commonly used scores, the new weighted score compared favorably, showing a better prediction than the unweighted score, Stanford and REGA while showing similar results albeit not substantially different than Virco's Virtual Phenotype.
- This analysis results in a weighting of mutations based on multivariate parametric or non-parametric methodology with adequate adjustments for background activity and proves to be an important advance for determining weighted mutation scores for protease inhibitors.
- The weighted score presented in this work is based on a sample of patients with a treatment history and a background ARV regimen that is representative of a fixed point in the HIV epidemic. It predicts response well and can be used in decision-making when deciding how to use tipranavir in the course of a patient's HIV therapy. It will need to be re-evaluated as additional data relating genotype to virologic response on tipranavir becomes available from currently ongoing studies in patient populations with differing treatment histories and differing background regimens.
Methods
Datasets
The base dataset consisted of all RESIST patients who had baseline genotype, were randomized and received at least one dose of tipranavir resulting in N=745 patients. This base dataset was randomly broken up into a score development dataset and an evaluation dataset consisting of roughly 75% and 25% of the base dataset (N=566 and N=179 for the development and the test datasets, respectively). The evaluation dataset was used for assessing the predictive accuracy of the mutation score and comparing it to other commonly used scores.
The RESIST study population was specific by design in excluding patients whose baseline genotype consisted of ≥3 substitutions at codons 33, 82, 84 and 90. Therefore it should be mentioned that some mutational patterns were not present in the dataset by design of the studies.
Score development
1. Mutations The initial list of mutations for consideration was restricted to the mutations in the existing TPV mutation score plus 5 mutations believed to increase susceptibility to TPV:
TPV score mutations - 10V, 13V, 20M/R/V, 33F, 35G, 36I, 43T, 46L, 47V, 54A/M/V, 58E, 69K, 74P, 82L/T, 83D, 84V [1].
Mutations associated with observed increased susceptibility to TPV - 24I, 30N, 50L/V, 54L, 76V.
2. Models Six models relating the mutations above to different response variables were run (Table 1).
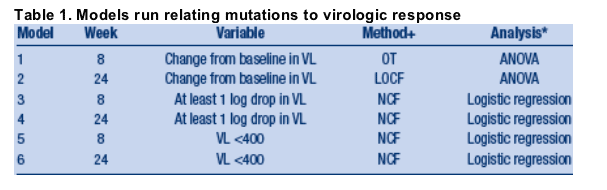
* All models adjusted for baseline CD4+ cell counts and OBR activity.
OT = On-Treatment - patients with missing values not included in analyses; LOCF = Last On-treatment Carried Forward;
NCF = Non-Completers considered Failures.
3. Cross-validation The test dataset was randomly divided into 10 subsets and each model was run 10 times, each time leaving out one of the subsets. This allowed both an assessment of the robustness of the model estimates (and hence weights) and also an independent, cross-validation estimate of the association of the weighted score with virologic response, after adjusting for baseline CD4+ cell count and background activity.
4. Determination of weights For each model (6 models) and run (10 runs), the weights for each mutation were formed as follows (note that for the logistic regression models, the log (odds ratios) were used rather than the parameter estimates but the methodology was the same):
1. The mutation with the largest absolute model estimate (max estimate = M) was given a weight of 10 (if "harms" virologic response) and -10 (if "helps" virologic response).
2. The other weights (W) were computed to keep the relationship of the parameter estimates (E) intact, with the integer value being retained (W = floor (10*(E / M))).
Table 2 shows the model estimates and odds ratios for run 3 (i.e. excluding the 10% of patients randomly selected into subset 3) and the corresponding calculation of weights for each model.
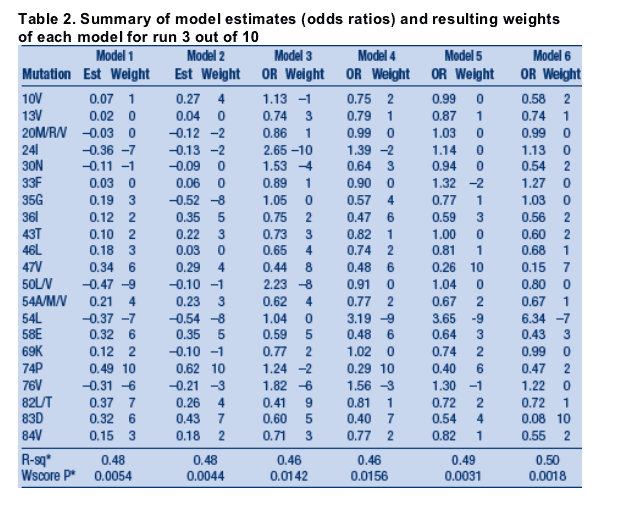
Est = Adjusted mean response without mutation - adjusted mean response with mutation (i.e. estimated amount of "harm" having the mutation will have on the virologic response - negative value implies the mutation is "helping" the response to drug).
OR = odds response / odds non-response.
* From regressing week 8 change in VL on baseline CD4+ cell count, background activity and the resulting weighted score using the N=70 patients contained in cross-validation subset 3. Wscore P is the p-value for showing the statistical significance of the weighted score.
5. Final weights Weights for each of the 6 models were determined by taking the median weight for the runs with the best fit on the cross-validation datasets. The final weight was determined by taking the median weight across the six models. The derivation of the final weights is shown in Table 3.
Determining clinical cut-offs The cut-offs for predicting a patient to be susceptible (S), partially susceptible (PS) or resistant (R) to TPV were determined based on the Area Under the Receiver Operator Curve (AUROC - the cut-points were chosen that resulted in the largest AUROC, after adjusting for baseline CD4+ cell count and background antiviral drug activity), a measure of prediction accuracy. The entire dataset of TPV/r patients in RESIST was used to determine the cut-offs.
Comparison to other scores
Roughly a quarter of the base dataset (N=179) was left out of the score development to provide an independent source to compare to other available mutation scores.
Comparing to other scores The tipranavir weighted score (TW) was compared to the tipranavir unweighted score [1] (TUW), the score from the Stanford website [2] (STAN), the score from Rega Institute for Medical Research version 7.0 (REGA) [3], and the score from the Virtual Phenotype (VP) methodology from Virco. Comparisons were made at weeks 8 and 24 by looking at:
1. Spearman correlation coefficients of weeks 8 (On-Treatment - missing data not replaced) and 24 (Missing values replaced by carrying forward the last observation on treatment) viral load changes from baseline.
2. Logistic regression models were run for each score with weeks 8 (at least a 1 log drop in viral load) and 24 (VL <50) virologic response as the dependent variable and predictors of TPV mutation score and OBR activity. The comparisons made between scores were the pvalue assessing statistical significance of each mutations score and AUROC to compare overall prediction accuracies.
Background Activity Scores
The development of the weighted score and (model-based) comparisons to other scores were all adjusted for the activity of the background regimen. The estimated activities for each patient were based on a novel approach developed by Hall et al. [4] that estimates the contribution of each drug in the Optimized Background Regimen using linear model-based approach accounting for the baseline resistance (using Virtual Phenotype) and historical use of each drug. Often, the activity of the drug in the background regimen, especially with the
NRTIs, is largely dependent on the previous pattern of use (due to archived resistance) rather than the baseline resistance. These adjustments prove to be much better at predicting response than a straight count of the number of active drugs in the OBR.
Results
Model fitting and determination of weights
The final weights are shown in Table 3 and can be divided in three categories as shown in Table 4. Some notes about the final weights:
- 33F, previously believed to be a key tipranavir mutation, receives a weight of 0 and thus has been removed from the weighted score.
- 84V, a major mutation for all PIs (receives a weight of 25 in the Stanford score), receives only a weight of 2 in the revised analyses.
- Mutations 54L, 50V and 76V, all representing major mutations contributing resistance to fosamprenavir and also to darunavir, predict improved response to tipranavir.
- Only 47V (14%), 54A/M/V (64%) and 58E (16%) were major mutations seen in over 10% of patients from the tipranavir development program. This is the reason such a small number of patients entering the RESIST trials were resistant to tipranavir providing further evidence of the unique resistance profile of tipranavir compared to the other protease inhibitors.
- TPV score mutations 13V and 69K, which were seen more frequently in non-clade B patients in the BI-sponsored trial of naive patients 1182.33 (13V: 17.2% B vs 48.4% non-B and 69K: 4.0% B vs. 66.2% non-B), both receive weights of 0 and are thus removed from the weighted score.
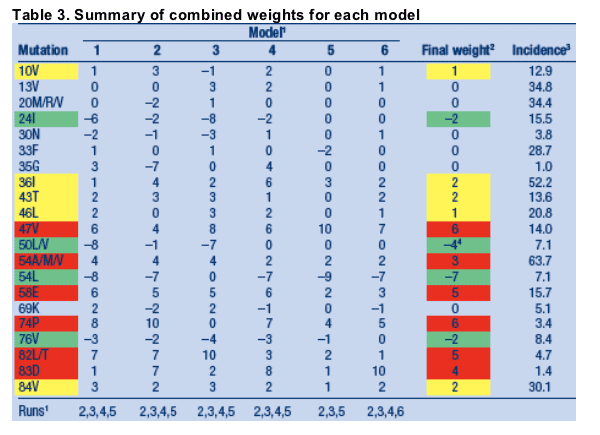
1 Weights for each model are arrived at by taking the median (rounded down) of all runs with significant p-values for the resulting weighted score when regressed against week 8 VL change from baseline, using the cross-validation datasets. The bottom row of the table shows which runs were used in the calculation of the median.
2 Median weight across models (also rounded down).
3 % of baseline isolates containing that mutation from the phase II/III TPV development program (excluding naive trial 1182.33).
4 Weight taken as the average of models 1 and 2 due to inconsistency of results and strong correlation with 50L/V and TPV phenotype.
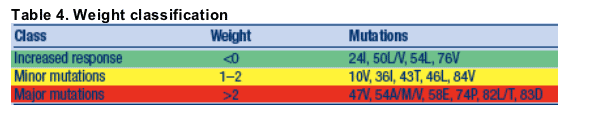
Performance and comparison to other scores
Cut-offs for the weighted score were determined using all RESIST TPV/r-treated patients. A lower cut-off of 3 and upper cut-off of 10 yielded the largest AUROC (78.5%) for predicting 24 week VL <50 copies/mL, after adjusting for baseline CD4+ cell count and OBR activity. The score levels with frequencies and numbers of responders are provided in Table 5. Also included is a summary of the response rates at each cut-point.
An increasing number of major mutations (47V, 54A/M/V, 58E, 74P, 82L/T, 83D) proves to be a good marker for the resulting resistance classifications. A cross-classification of number of major mutations with clinical interpretation of the weighted score is included in Table 6. These data suggest the following regarding the interpretation of the tipranavir weighted score:
- You need at least 2 major mutations to be resistant to tipranavir and with ≥3 full resistance was seen in all isolates available.
- With ≦1 of the major mutations, TPV/r is likely to retain partial susceptibility and with no major mutations nearly all isolates retained full susceptibility to TPV/r.
The results of the comparisons to other scores using the independent dataset are provided in Table 7. The TW and VP both predict better than the other three scores at both weeks 8 and 24, with VP having the highest correlation with viral load decline (R = 0.25) and prediction accuracy (71.0%) at week 8 and TW being the best at week 24 (R = 0.35 and AUROC = 71.2%). It makes sense that these two scores would perform the best since they are both based on a similar methodology, that being a linear-model based approach to estimate the effects of each mutation. Slight differences are that VP is developed on a much larger
database and is designed to predict phenotype, whereas TW is designed to predict virologic response.
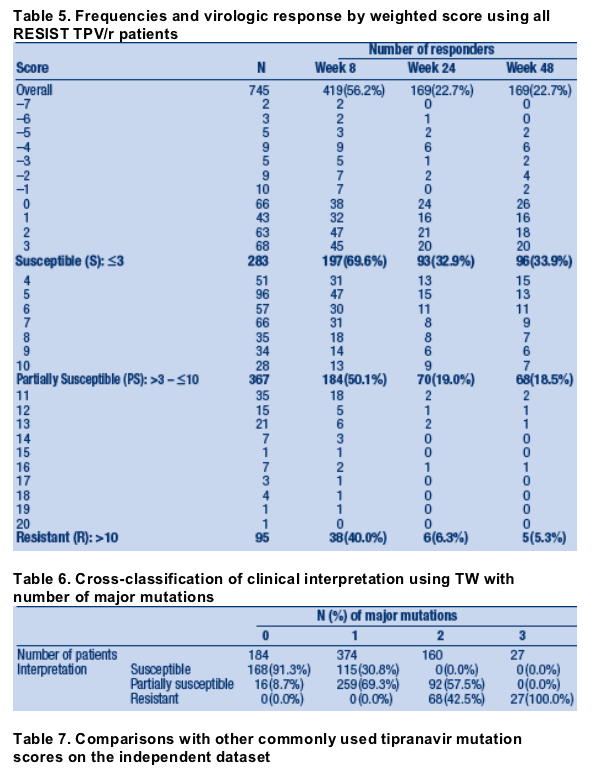
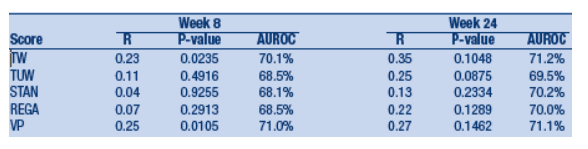
R = Spearman correlation coefficient of viral load change from baseline and the respective score.
P-value = Significance level for each weighted score from a logistic regression model, adjusted for OBR activity and the weighted score.
AUROC = Area Under the Receiver Operator Curve measuring the prediction accuracy from a logistic regression model adjusting for OBR activity. Week 8 predictions are of a 1 log decline in viral load from baseline and week 24 are of getting below detection (VL <50).
Discussions
- It is clear that in determining a mutation score, a two-step approach is needed that first reduces the total number of mutations in the model by a univariate procedure and then applies a multiple selection procedure to arrive at a combination that best predicts response [6]. The first step was already done to identify the TUW score so that we began with the pre-defined set of tipranavir score mutations plus a handful of mutations believed to be associated with
hypersusceptibility or increased response. When additional data relating genotype to virologic response on tipranavir becomes available from currently ongoing studies with TPV, the score will be reviewed.
- Part of the score revision analysis plan can be a comparison of the methodology used in this work with other weight determinations, including Flandre's resampling methodology [5], a purely non-parametric approach, recursive partitioning modified to account for weighting, or other model-based approaches albeit with different methods to arriving at the weights from model estimates, including the impact of varying the maximum weight on score performance.
References
1. Baxter JD et al. Journal of Virology 2006; 80:10794-801.
2. Stanford University HIV Drug Resistance Database
3. Algorithm for the use of genotypic HIV-1 resistance data, Rega v7.0 , 6 March 2007
4. Hall DB, et al. 11th European AIDS Conference / EACS, October 24 - 27 2007, Madrid, Spain (Poster # P4.3/71)
5. Flandre P, et al. XV International HIV Drug Resistance Workshop, June 7-11 2005, Quebec City, Canada (Abstract # 167)
6. F Brun-Vezinet, et al. Antiviral Therapy 2004; 9: 465-478
7. Schapiro JM, et al. 11th European AIDS Conference / EACS, October 24 - 27 2007, Madrid, Spain (Poster # P3.4/09)
|
|
|
 |
 |
|
|