|
Prediction of Incident Diabetes Mellitus in Middle-aged Adults
|
|
|
The Framingham Offspring Study
Peter W. F. Wilson, MD; James B. Meigs, MD, MPH; Lisa Sullivan, PhD; Caroline S. Fox, MD, MPH; David M. Nathan, MD; Ralph B. DfAgostino, Sr, PhD
Arch Intern Med. May 30, 2007;167:1068-1074.
Easy to identify Predictors of diabetes onset in white adults in impaired fasting glucose 100-126 mg/dL most important predictor (Odds Ratio 7.25), BM! >30 (OR 2.50), HDL-C <40 mg/dL in men or <50 mg/dl in women (OR 2.57), triglyceride level >150 mg/dl (OR 1.78), blood pressure >130/85 mm Hg or receiving therapy (OR 1.65), waist circumfrance >102 c, in men or >88 cm in women, parental history of diabetes (OR 1,76).
We estimated the 7-year risk of T2DM in middle-aged whites...In summary, we found that complex models are not needed to predict T2DM and that information from a typical clinic visit adds to T2DM prediction beyond personal awareness of diabetes risk factors. The simple clinical model we developed should be tested in other population samples to validate our approach, as has been done for prediction of coronary heart disease events.... An impaired fasting glucose finding (10 points), a BMI of 30.0 or greater (5 points), and a low HDL-C level (5 points) had the greatest effects in the point scores, and successively smaller effects were evident for a positive parental history (2 points), a triglyceride level greater than 150 mg/dL (>1.7 mmol/L) (2 points), a BMI of 25.0 to 29.9 (2 points), and elevated blood pressure (2 points)...."
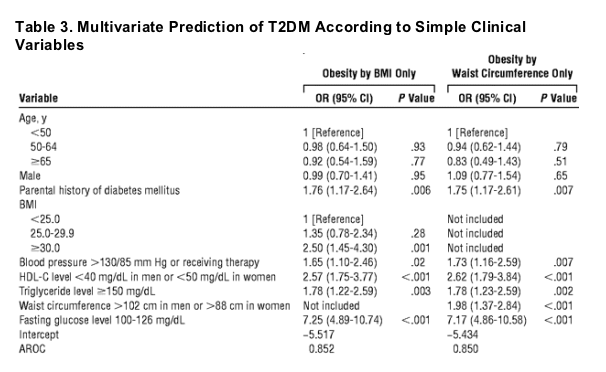
ABSTRACT
Background Prediction rules for type 2 diabetes mellitus (T2DM) have been developed, but we lack consensus for the most effective approach.
Methods We estimated the 7-year risk of T2DM in middle-aged participants who had an oral glucose tolerance test at baseline. There were 160 cases of new T2DM, and regression models were used to predict new T2DM, starting with characteristics known to the subject (personal model, ie, age, sex, parental history of diabetes, and body mass index [calculated as the weight in kilograms divided by height in meters squared]), adding simple clinical measurements that included metabolic syndrome traits (simple clinical model), and, finally, assessing complex clinical models that included (1) 2-hour post-oral glucose tolerance test glucose, fasting insulin, and C-reactive protein levels; (2) the Gutt insulin sensitivity index; or (3) the homeostasis model insulin resistance and the homeostasis model insulin resistance -cell sensitivity indexes. Discrimination was assessed with area under the receiver operating characteristic curves (AROCs).
The population sample included 3140 men and women who attended the fifth clinic examination of the Framingham Offspring Study in the mid-1990s. This population sample is 99% white and non-Hispanic.
Results The personal model variables, except sex, were statistically significant predictors of T2DM (AROC, 0.72). In the simple clinical model, parental history of diabetes and obesity remained significant predictors, along with hypertension, low levels of high-density lipoprotein cholesterol, elevated triglyceride levels, and impaired fasting glucose findings but not a large waist circumference(AROC, 0.85). Complex clinical models showed no further improvement in model discriminations (AROC, 0.850-0.854) and were not superior to the simple clinical model.
Conclusion Parental diabetes, obesity, and metabolic syndrome traits effectively predict T2DM risk in a middle-aged white population sample and were used to develop a simple T2DM prediction algorithm to estimate risk of new T2DM during a 7-year follow-up interval.
We developed a point score system to estimate diabetes risk using the intercept and the beta coefficients of the simple clinical model that used BMI as the adiposity measure. This approach allows manual estimation of the 8-year risk of developing diabetes, as shown in Table 6. An impaired fasting glucose finding (10 points), a BMI of 30.0 or greater (5 points), and a low HDL-C level (5 points) had the greatest effects in the point scores, and successively smaller effects were evident for a positive parental history (2 points), a triglyceride level greater than 150 mg/dL (>1.7 mmol/L) (2 points), a BMI of 25.0 to 29.9 (2 points), and elevated blood pressure (2 points). The age categories and sex were not related to development of diabetes, and no age or sex variables were included in the point calculations. By using the point score, we determined that 63.8% of the sample had a less than 3% risk, 20.7% had a 3% to 10% risk, and 15.6% had a greater than 10% risk of incident diabetes during an 8-year interval. The AROC for the point score prediction was 0.850.
COMMENT
The principal finding of this study is that some information beyond personal awareness of diabetes risk factors is important to determine risk of T2DM, but complex models are not needed. We started with a personal model that included information generally available to persons before a clinic visit with a physician. The simple clinical model that included common metabolic traits efficiently identified subjects at elevated risk for T2DM diabetes, suggesting that clinical screening adds value beyond screening using personal knowledge alone. Consideration of 3 complex clinical models showed negligible improvement in assessment of T2DM risk over and above the simple clinical model.
Others who have reported using questionnaire data in cross-sectional studies to identify persons with undetected T2DM or to increase the yield of glucose testing have found that greater age, higher BMI, and ethnicity were especially important predictors.22-23 Where investigated, hypertension history, physical activity, and parental history of diabetes have been shown to be predictive of an abnormal OGTT result.24
To test the utility of questionnaire data, investigators in Cambridge, England, undertook an external validation study and found that age, sex, BMI, use of corticosteroids and antihypertensives, smoking, and parental history of diabetes mellitus were predictive elements of prevalent T2DM with an AROC of 0.80.25 Other longitudinal studies have used the Cambridge risk score prognostically to track individuals for deterioration in glycemic status, shown by a hemoglobin A1c level of greater than 7.0%, and have shown good success in predicting such deterioration in glycemia with an AROC of 0.74 in a British cohort.26
Others have examined these factors as determinants of T2DM in cross-sectional and longitudinal studies, including the San Antonio Heart Study,27 Insulin Resistance Atherosclerosis Study,28-29 Rancho Bernardo,30 and Munster31 cohorts. An analysis of the Atherosclerosis Risk in Communities data, which included more than 7900 adults aged 45 to 64 years, showed a high degree of model discrimination of future T2DM cases during follow-up when the National Cholesterol Education Program metabolic syndrome variable count of 0 to 5 was used in the analysis (AROC, 0.78).32 The authors concluded that rules based on the metabolic syndrome are reasonable alternatives for estimating risk for T2DM,32 similar to a recent report from the Framingham experience33 in which the National Cholesterol Education Program metabolic syndrome trait count was highly related to a greater risk for developing T2DM.
The traits that constitute the metabolic syndrome are especially important in the determination of risk for T2DM.33 The simple clinical models presented in Table 3 show that each of the metabolic syndrome traits is highly associated with the development of T2DM, and T2DM risk varies considerably across the variables. This supports a predictive approach wherein each variable should be used individually.
Because the simple clinical approach represented an easy and effective approach to estimate risk for the development of incident diabetes, we transformed the simple clinical model that used BMI into a point score that can be used in the office setting. As has been the case for prediction of coronary heart disease,2, 21 the availability of a simple clinical tool to estimate disease risk should improve the prediction of events and enhance prevention strategies.
Others have undertaken to predict or identify risk of diabetes mellitus with a variety of approaches. The American Diabetes Association prediction algorithm is based on the experience of the second National Health and Nutrition Examination Survey.4 The American Diabetes Association model used a decision tree, and a point score was developed to estimate risk. The authors reported an AROC of 0.78 with this approach.4 The key variables in that formulation were the birth of a child with macrosomia, obesity, sedentary lifestyle, and a parental history of diabetes mellitus. Their approach used self-reported personal information that identified individuals cross-sectionally and did not predict incident diabetes over time.
San Antonio researchers have developed a diabetes prediction rule that included simple clinical variables.5 Their model predicted the development of T2DM during a 7.5-year interval, and the key prediction variables were age, sex, Mexican American ethnicity, fasting plasma glucose level, systolic blood pressure, HDL-C level, BMI, and parental history of diabetes. In South Texans of Hispanic descent, the absolute risk for T2DM is much greater than in white subjects from suburban Boston or in Europe; the discriminatory capacity of their approach was high (AROC, 0.843-0.845), and the metabolic syndrome variables included were important diabetes predictors. Just as in the present Framingham analyses, more sophisticated measures, such as postchallenge plasma glucose level, did not add to the discriminatory capacity of more simple models. The utility of the San Antonio model has been tested in a German cross-sectional cohort and in a Japanese American prospective cohort.6 In the latter setting, the authors reported that the multivariate clinical model was better than the fasting glucose level for predicting development of T2DM after 5 or 6 years, but not after 10 years; the clinical model's predictive capability was similar to the predictive capability of the fasting or 2-hour glucose level in older Japanese Americans.
Investigators from Finland developed a diabetes risk score and predicted T2DM during 5 years of follow-up in a middle-aged population sample that identified cases by initiation of diabetes medications.34 They found that age, BMI, waist circumference, history of blood pressure therapy, high blood glucose level, physical activity, and dietary components were predictive of events. This approach, with separate identification and weighting of metabolic factors, most closely parallels the results we obtained with the simple clinical model, but they did not use a formal OGTT at the beginning of their study.
In summary, we found that complex models are not needed to predict T2DM and that information from a typical clinic visit adds to T2DM prediction beyond personal awareness of diabetes risk factors. The simple clinical model we developed should be tested in other population samples to validate our approach, as has been done for prediction of coronary heart disease events.21, 35-36
METHODS
The population sample included 3140 men and women who attended the fifth clinic examination of the Framingham Offspring Study in the mid-1990s. This population sample is 99% white and non-Hispanic. The baseline examination included information on medication use and self-reported parental history of T2DM, defined as diabetes in one or both natural parents.11-12 The physical examination included blood pressure measured in the sitting position, height and weight measurements, and waist circumference determined at the umbilicus with the subject standing. Body mass index (BMI) was calculated by dividing the weight in kilograms by the height in meters squared.
Subjects were examined after an overnight fast and had a 2-hour oral glucose tolerance test (OGTT). Persons with a history of diabetes mellitus, who used oral hypoglycemic medications or insulin, or who had a baseline fasting plasma glucose level greater than 126 mg/dL (>7.0 mmol/L) or a baseline post-OGTT plasma glucose level greater than 200 mg/dL (>11.1 mmol/L) were categorized as having diabetes and were not included in this study. An OGTT 2-hour glucose level of 140 to 200 mg/dL (7.8-10.9 mmol/L) defined impaired glucose tolerance. Other laboratory measurements included levels of fasting and 2-hour OGTT insulin (determined using a commercially available assay [DPC Coat-a-Count; Diagnostics Products Corporation, Los Angeles, Calif]), total cholesterol, high-density lipoprotein cholesterol (HDL-C), triglycerides, and C-reactive protein (determined using a commercially available assay [Hemagen Diagnostics Inc, Waltham, Mass]), as previously described.11, 13
More sophisticated indexes of glucose and insulin control included calculation of the homeostasis model (HOMA) insulin resistance index, the HOMA -cell index as a measure of reserve pancreatic insulin production, and the Gutt insulin sensitivity index, which includes body weight and OGTT glucose and insulin information and is similar to a glucose disposition index.14-16 Persons were categorized according to the presence or absence of the metabolic syndrome traits described by the National Cholesterol Education Program Adult Treatment Panel III criteria.17 Participants with a blood pressure level of 130/85 mm Hg or higher or receiving treatment for hypertension were considered to have elevated blood pressure; those with a fasting glucose level of 100 to 126 mg/dL (5.4-6.9 mmol/L) were considered to have fasting hyperglycemia; a waist circumference greater than 102 cm in men or more than 88 cm in women was considered increased; a fasting triglyceride level of 150 mg/dL or greater (1.7 mmol/L) was considered hypertriglyceridemia; and an HDL-C level less than 40 mg/dL (<0.9 mmol/L) in men or less than 50 mg/dL (<1.2 mmol/L) in women was considered low.18-19
Participants were followed up from baseline to the sixth (1995-1998) and seventh (1998-2001) Framingham Offspring Study examinations for an average follow-up of 7 years. We used the examination visit date that a new case of diabetes was identified as the date of diagnosis; otherwise follow-up was censored at the last follow-up (examination 6 or 7) for participants remaining nondiabetic. Participants were characterized as developing new diabetes during follow-up if they (1) started receiving oral hypoglycemic agents or insulin or (2) had a fasting glucose level of 126 mg/dL or greater (>/=7.0 mmol/L) at 1 of the follow-up Framingham Offspring Study examinations conducted 4 and 7 years after the baseline examination.
Statistical analyses included a series of logistic regression models to predict incident diabetes, using the odds ratio and 95% confidence intervals to estimate relative risk. Alternate analyses using Cox proportional hazards models that accounted for interval censoring gave essentially identical results; only logistic regression results are presented. The rationale for separate models to estimate T2DM risk for diabetes was predicated on evaluation of 3 major levels of health information. The first level, the personal model, was based on information known to an individual without seeking medical advice. The second level, the simple clinical model, was based on personal model variables plus information typically available at a clinic visit with a physician. The third level, a set of complex clinical models, incorporated simple clinical model covariates plus information that is available only with more detailed clinical testing, including data from an OGTT and measurement of insulin levels and inflammatory markers.
Our regression models sequentially included the personal, simple clinical, and more complex clinical models, with evaluation of the discriminatory capability of the models using the C statistic, or the area under the receiver operating characteristic curve (AROC). Between-model comparisons were evaluated by ranking participant risk by decile and performing a X2 analysis on the estimates as per Hosmer and Lemeshow.20 Score sheets to estimate absolute risk for the outcome were derived from the beta coefficients of the multivariate logistic regression analysis, as described previously.2, 21
|
|
|
|
|
|
|