 |
 |
 |
|
Innovative Machine Learning Tool Predicts
Who Might Have Non-Alcoholic Steatohepatitis
|
|
|
BOSTON - Data from a new study presented this week at The Liver Meeting® - held by the American Association for the Study of Liver Diseases - found that a machine-learning tool could successfully predict the risk of having no n-alcoholic steatohepatitis (NASH) among patients with co-existing diseases.
NASH, the advanced form of non-alcoholic fatty liver disease (NAFLD), is often underdiagnosed, making the identification and validation of an accurate screening tool highly valuable in clinical practice.
Researchers at Novartis Pharma AG, Basel, Switzerland; ZS Associates in New Jersey and the University Medical Center Mainz in Germany conducted the study with an objective to develop and validate a machine learning algorithm to predict the risk of having NASH using non-invasive, regularly collected clinical parameters available in two real-world databases. The study's co-authors conducted exploratory analysis, feature extraction, model training and parameter tuning on the NAFLD Adult Database of the National Institute of Diabetes, Digestive and Kidney Diseases (NIDDK) of the National Institutes of Health, which includes data on people with confirmed NASH and non-NASH NAFLD.
The researchers tested the best-performing model from the NIDDK database on the Optum de-identified Electronic Health Record (EHR) dataset. Performance measures such as area under the curve (AUC), diagnosis sensitivity, specificity and overall accuracy were analyzed. This was an extreme gradient-boosting model, XGBoost, with an AUC of 0.82, which included 14 clinical variables. The AUC of the model was 0.76 in the Optum EHR database.
Using the XGBoost 14-parameter model on patients in the Optum EHR, the researchers were able to predict up to 29,000 additional, non-identified NASH patients per 100,000 people in the cohort. The researchers also developed a simplified model with five clinical variables which showed a slightly lower performance, with an AUC of 0.80 in the NIDDK and 0.74 in Optum EHR on the same patient cohort.
"This is an innovative machine learning algorithm developed to help identify potential NASH patients in large datasets based on various clinical variables," says Jörn M. Schattenberg, MD, head of metabolic liver disease and the translational hepatology research laboratory, Department of Gastroenterology and Hepatology, Johannes Gutenberg-Universitat Mainz. "The algorithm could be used to support earlier screening and management of potential NASH patients, as well as support recruitment of future clinical trials. Future development options, such as the integration into medical records software, could also be considered."
Dr. Schattenberg will present these findings at AASLD's press conference in Room 210 at the Hynes Convention Center in Boston on Saturday, Nov. 9 from 4 - 5:30 PM. The study entitled "AN INNOVATIVE TOOL BASED ON MACHINE LEARNING TECHNIQUES PREDICTS NASH PATIENTS IN REAL-WORLD SETTINGS," will be presented on Monday, Nov. 11 at 10:30 AM in the Constitution Ballroom. The corresponding abstract (number 0190) can be found in the journal, HEPATOLOGY.
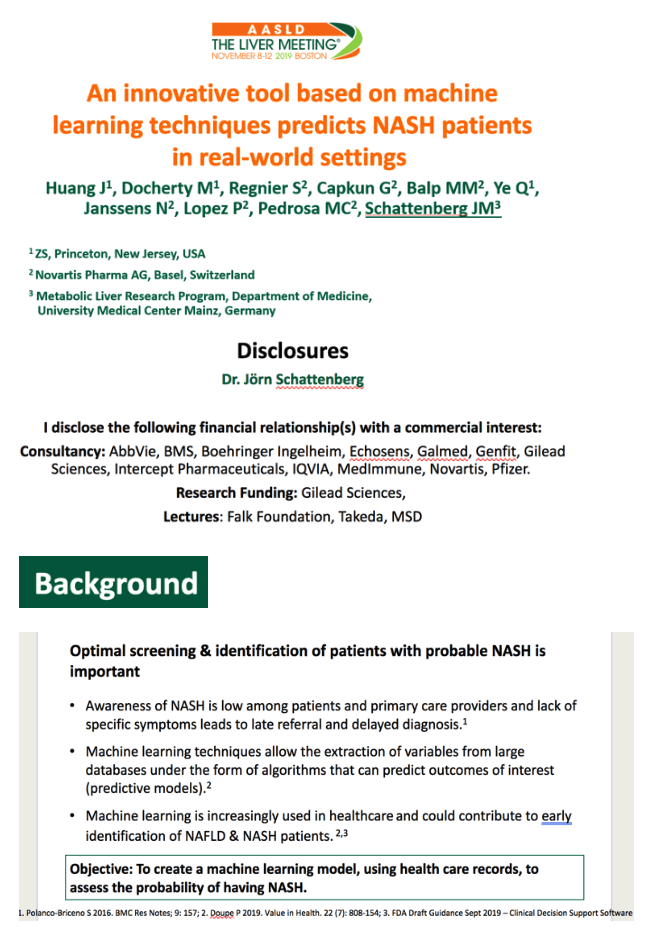
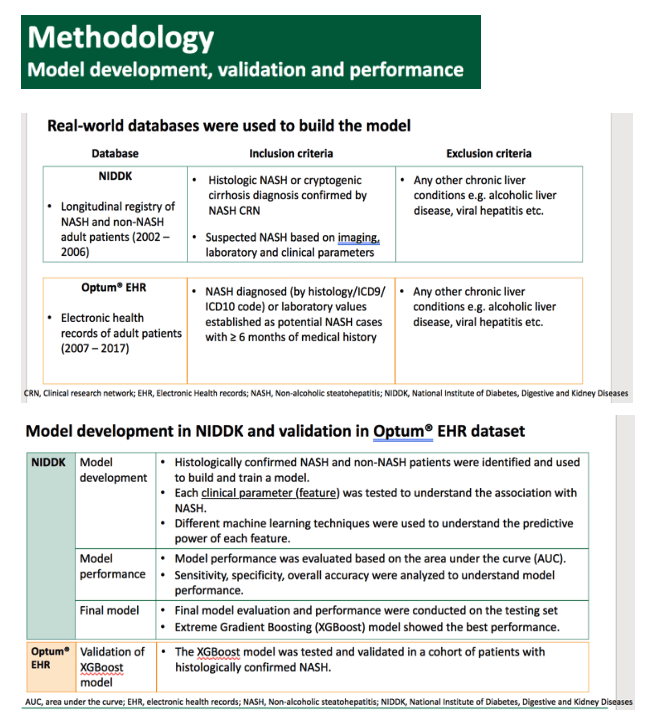
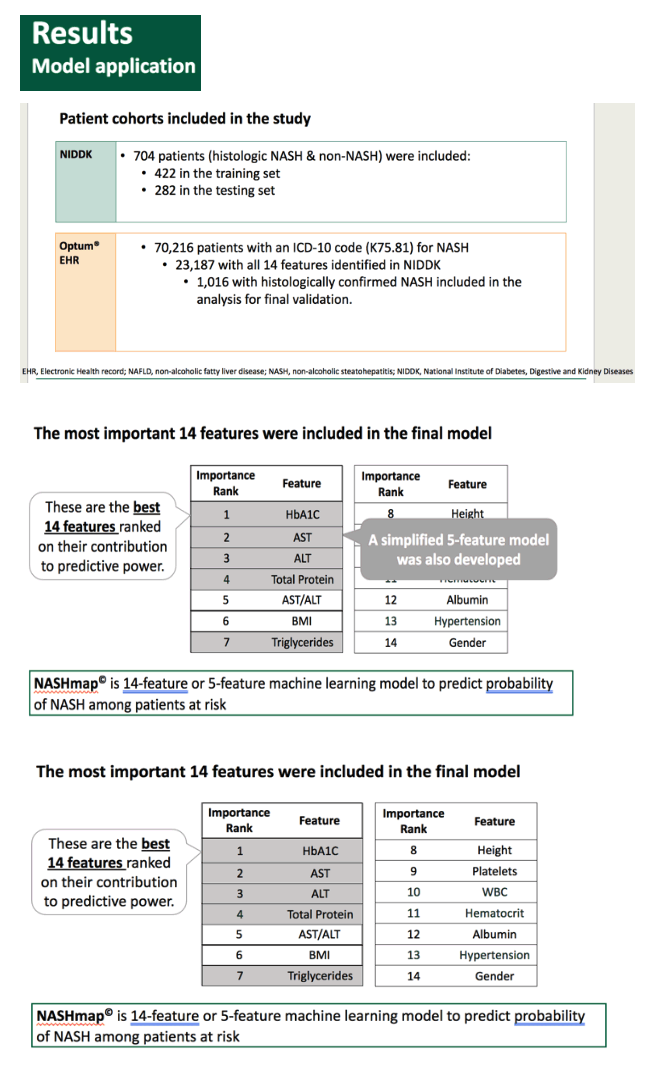
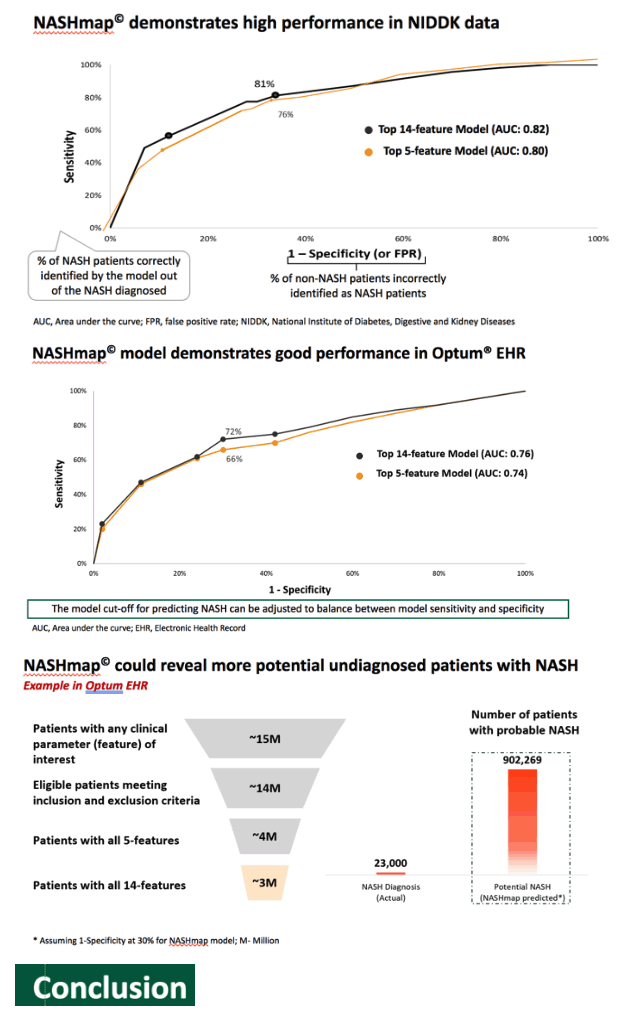
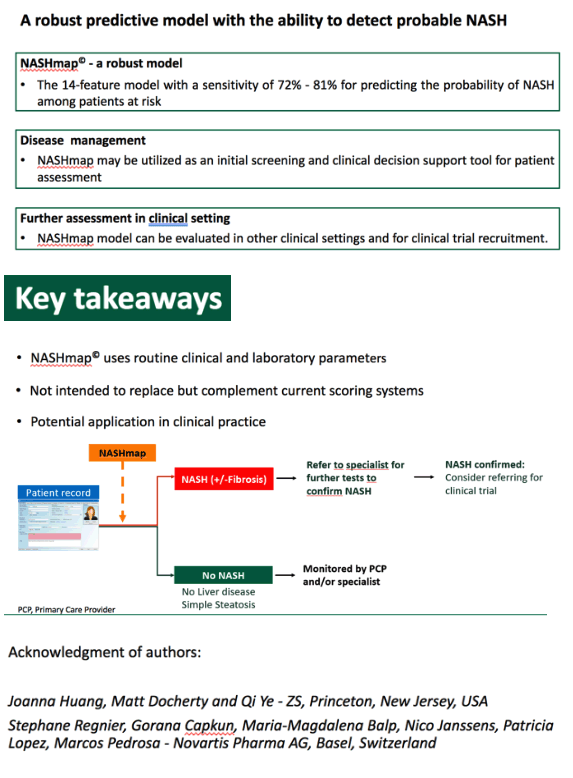
|
|
|
 |
 |
|
|