 |
 |
 |
|
POTENTIAL COST-EFFECTIVENESS OF A MACHINE LEARNING ALGORITHM
TO IDENTIFY UNDIAGNOSED HEPATITIS C PATIENTS IN THE UNITED STATES
|
|
|
AASLD 2021 Nov 12-15
Download the PDF here
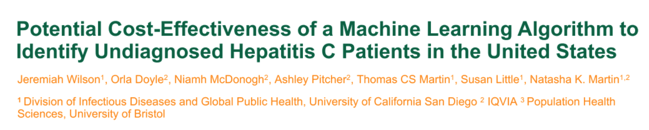
published study: pdf attached
Finding undiagnosed patients with hepatitis C infection: an application of artificial intelligence to patient claims data - June 2020 Scientific Reports - "Important risk factors identified by the algorithm included age, treatments for pain and arthritis as well as recognised HCV symptoms such as joint pain and sore muscles. IV drug use was also an important factor, associated with over a four-fold increase in the risk of HCV. For HCV specifically, the authors of this study previously conducted a feasibility study into the use of medical history and prescription data for predicting the diagnosis of HCV with models achieving a precision of 72% at a recall of 50%19. In this study, a suite of machine learning algorithms was developed to predict diagnosis or treatment of HCV in a universe of approximately 10 million patients using medical claims and prescription data captured between 2010 and 2016. In Fig. 1, the HCV patient journey is illustrated using the average time between the date of first occurrence of events and the index date across HCV patients. The HCV patient journey showed that patients experienced known symptoms of HCV (joint pain, abdominal pain, malaise/fatigue, fibromyalgia) on average 2-3 years prior to their diagnosis. Treatment with NSAIDs, systemic steroids, opioids occur 3-4 years earlier indicating that these patients are seeking treatment for these symptoms prior to receiving their diagnosis for HCV. Patients are also undergoing several diagnostic test procedures close to the time of their diagnoses. Relative risk curves for the top three most important features and the count of unique selected treatments patients received are displayed in Fig. 4. Patients who were prescribed NSAIDs up to once per year had a two-fold increase in risk of HCV compared with patients who had not received treatment with NSAIDs whereas patients with more frequent use of NSAIDS (>1 per year) had a similar or reduced risk of HCV. A modest increase in risk was observed for patients aged between 50 and 60 years (relative risk of 1.4) as compared to patients aged between 20 and 40 years. Risk of HCV increased by five-fold in patients with 10 or more instances of IV drug use as compared to patients without evidence of IV drug use. Patients with up to five unique treatments were observed to have a three-fold increase in risk of HCV as compared to patients without these selected treatments. HCV patients were identified as those with a diagnosis code or treatment for HCV. This latter condition based on treatment is necessary given that not all diagnoses are observed in the data. The list of HCV diagnosis codes and HCV treatment products are listed in Tables S1 and S2, respectively. The index date for HCV patients was defined as a medical claim or prescription event occurring prior to the first observed date of diagnosis or treatment for HCV, ensuring only pre-diagnosed attributes were used. The lookback date for HCV patients was defined as the earliest observed event where a patient had activity in both prescription and medical claims databases. An observation window was defined as a time period between the index date and the lookback date... https://www.nature.com/articles/s41598-020-67013-6
Jeremiah Wilson1, Natasha Martin2, Annie Son3, Susan LIttle1, Thomas Martin1, Niamh Mcdonogh4, Ashley Pitcher4 and Orla Doyle4, (1)University of California, San Diego, (2)Medicine, University of California San Diego, (3)Gilead Sciences, (4) Iqvia
Background: Despite new U.S. Centers for Disease Control and U.S. Preventative Services Taskforce guidelines for universal screening of all adults aged 18 and older for hepatitis C virus (HCV), many remain undiagnosed, and even more so due to the COVID-19 pandemic. Machine learning (ML) algorithms are potentially effective at improving the HCV care cascade. We evaluate the potential cost-effectiveness of a ML algorithm to identify undiagnosed HCV patients in care, using data from an algorithm developed based on U.S. ambulatory electronic medical records (EMR).
Methods: The algorithm was trained using 16M patients from U.S. ambulatory EMR data across primary and specialty care from 2015-2020. The algorithm was developed to identify undiagnosed HCV patients in a 12-month prediction window using medical history from a 24-month lookback with a 1-month offset. Algorithmic sensitivity for various levels of Positive Predictive Value (PPV) was assessed on an independent cross section of the data. A HCV natural history Markov model was used to evaluate the cost-effectiveness of the ML algorithm compared to status quo screening used to identify patients over the training data period (risk-based and birth-cohort screening, PPV ~2%). We compared the status quo to scenarios with the machine learning algorithm at different sensitivity levels (5-100%). We identified optimal algorithm sensitivity which maximized health (measured in quality-adjusted life years, QALYs) while staying under a willingness-to-pay threshold of USD$100,000/QALY gained. Based on the algorithm's performance on EMR data, we assumed patients were diagnosed 6.5 months sooner than status quo.
Results: The ML algorithm was cost-effective (ICER<$100k/QALY gained) in identifying undiagnosed HCV patients for sensitivity levels of up to 40% (Figure 1). The optimal sensitivity level of 40% (PPV 0.17%) resulted in incremental costs of $96.90 [95% CI 77-118] and incremental QALYs of 0.0011 [95% CI 0.0008-0.0014], and produced a mean ICER of $92,245/QALY gained.
Conclusion: ML algorithms to identify undiagnosed HCV patients could be cost-effective in the U.S., so evaluating real-world effectiveness is warranted. As algorithms can be tuned to a desired tradeoff between PPV and sensitivity, economic modeling can inform this tradeoff.
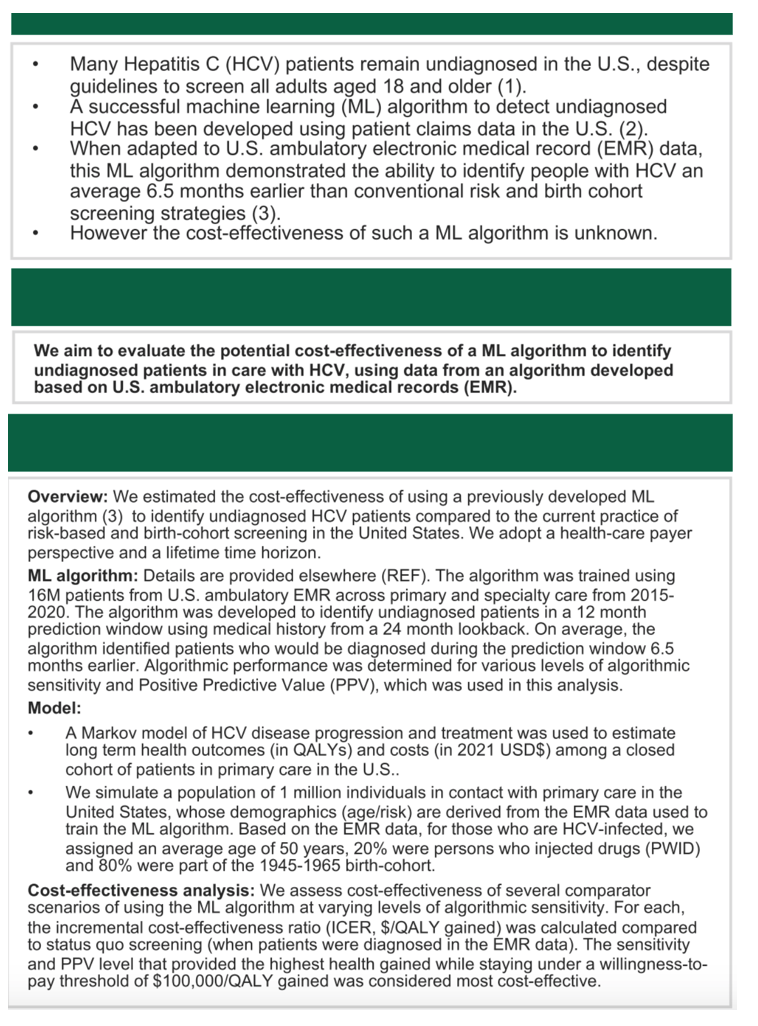
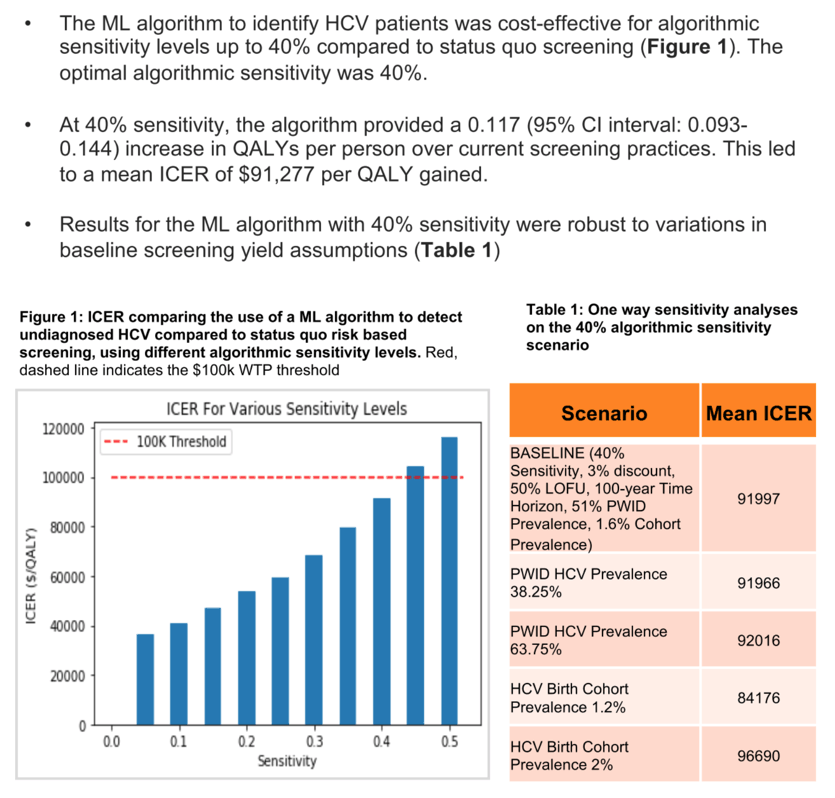
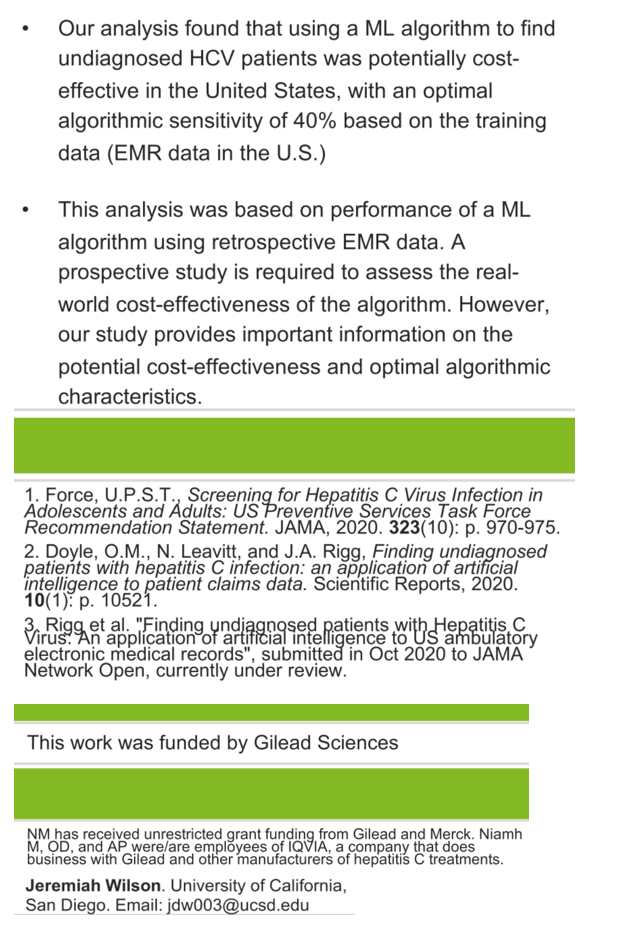
|
|
|
 |
 |
|
|