 |
 |
 |
|
EHR HCV Screening - OPTIMIZING HIGH- RISK HEPATITIS C VIRUS (HCV) IDENTIFICATION BY THE INCLUSION OF STRUCTURED, SEMI- STRUCTURED AND FREE- TEXT ELECTRONIC HEALTH RECORD DATA
|
|
|
AASLD 2022 Nov 4-8

program abstract
Background: New cases of HCV in the US have tripled from 2005- 2015, primarily due to the rise in opioid use. Despite efforts to improve targeted risk- based testing, evaluation of the CDC- defined HCV-related risk factors are not consistently performed as part of routine care, rendering risk- based testing susceptible to clinician bias and missed diagnoses. This work aims to use natural language processing (NLP) and machine learning to identify patients who are at high risk for HCV infection, new or re- infection.
Methods: Our study uses data between 1/1/20 - 10/31/20 from a large academic health system in the mid- Atlantic US. Models were developed and validated to predict patients with HCV infection (detectable RNA or reported HCV diagnosis). We extracted structured, semi- structured, and free-t ext predictors from the EHR, based on published literature and clinical expertise. We used a least absolute shrinkage and selection operator (LASSO) logistic regression to predict HCV infection. One model used only structured predictors, "structured- only model"; an expanded model used structured, semi- structured and free-text notes predictors, "full-feature model." Models were evaluated using the C- statistic; analysis was done in Python 3.9. We used ten-fold cross- validation to evaluate the generalizability of the models and minimize overfitting and present the average and 95% confident interval (CI).
Results: There were 3564 unique patients, 487 with HCV infection. The average C- statistics on the structured- only and full-f eature models for all the patients were 0.872 (95% CI: 0.863- 0.881) and 0.873 (95% CI: 0.842- 0.904), respectively. NLP was able to identify six risk factors not consistently coded in structured elements (Table 1): 74 patients had a history of incarceration, none had the associated ICD10 Z65.1; ten had a needlestick injury, one had ICD10 W46.
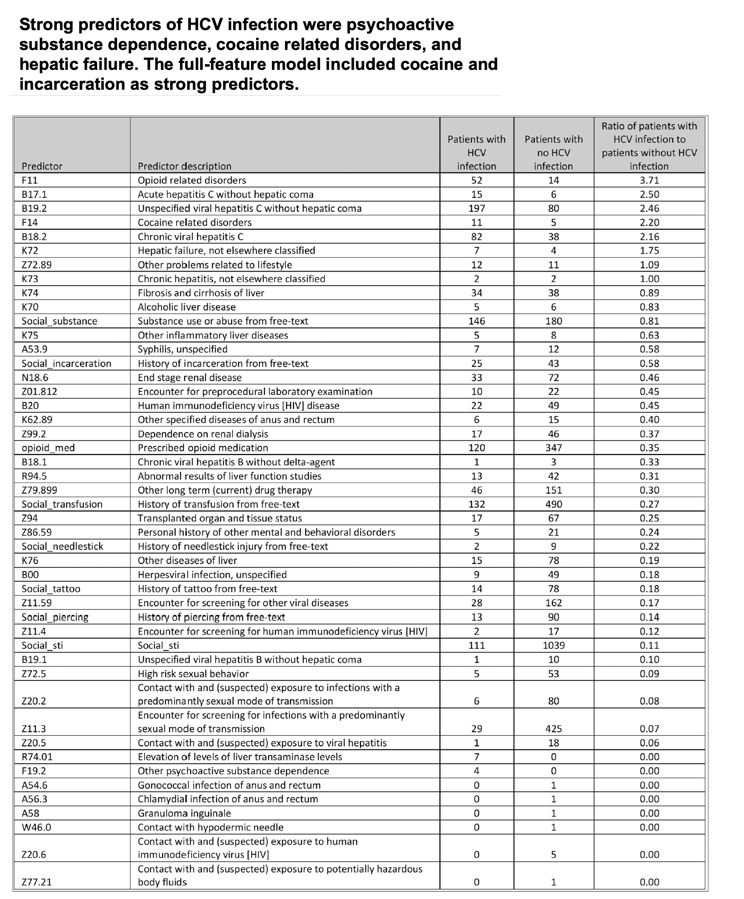
Conclusion: The full-feature model performed slightly better than the structured- only model; however NLP use was able to extract six risk factors not readily available in structured elements. Development of this prediction model will be used in a clinical decision support alert, in addition to universal screening, for providers to test and re-test high-risk patients for HCV, thus contributing to HCV micro- elimination.
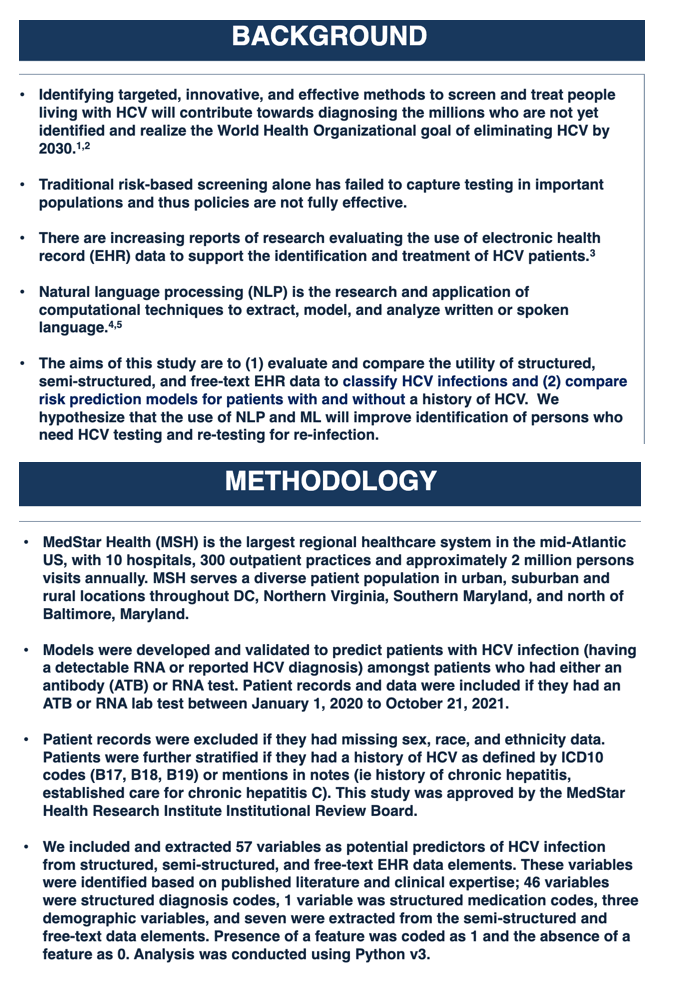
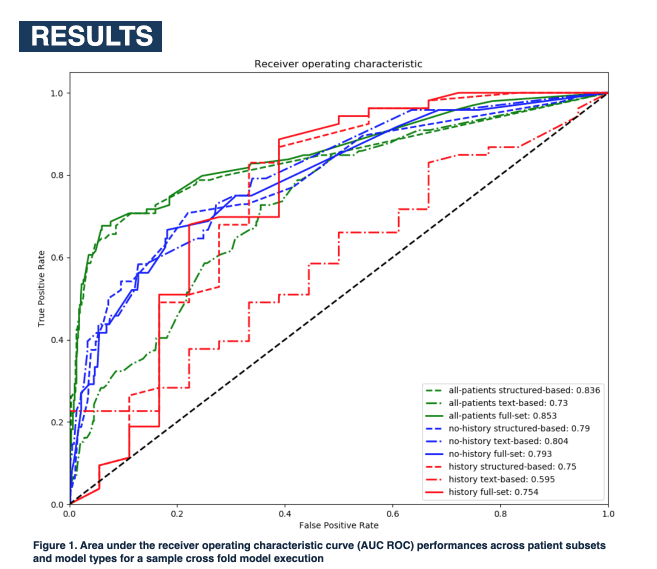
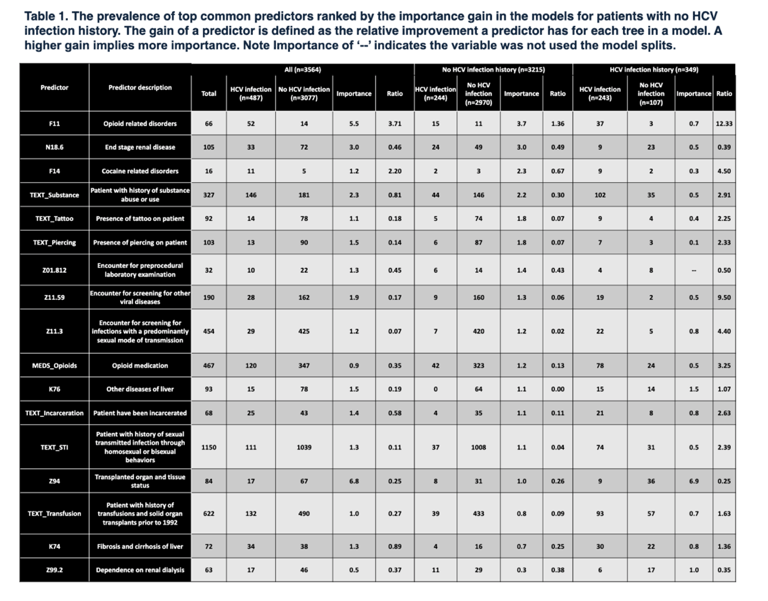
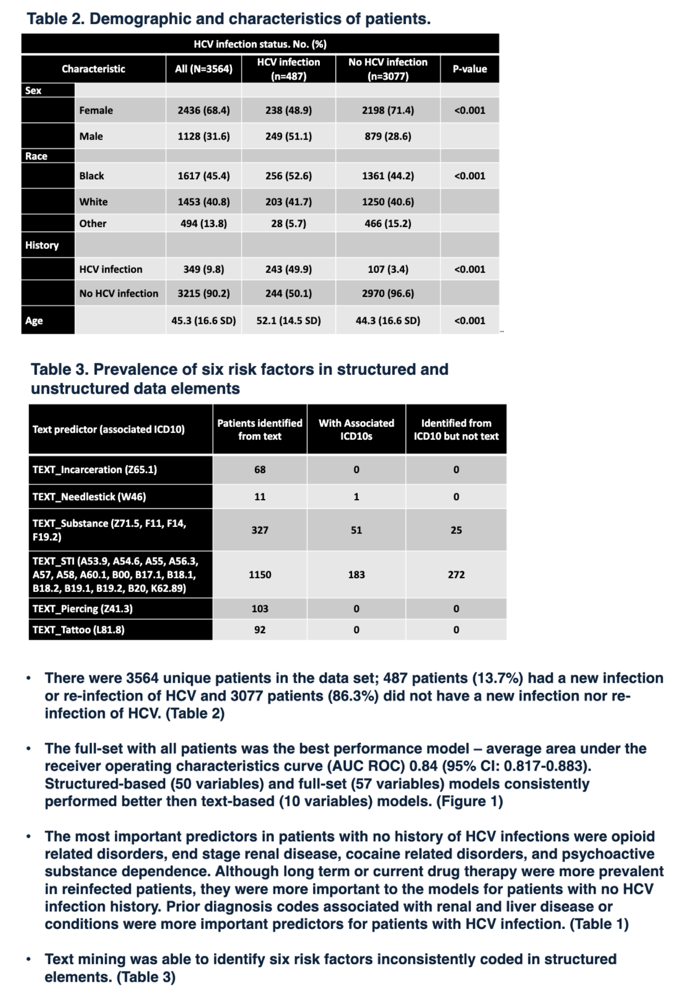
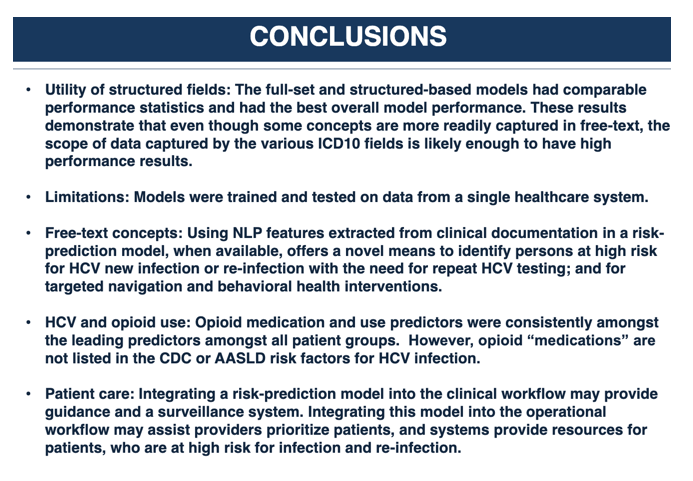
|
|
|
 |
 |
|
|