|
Patient preferences and assessment of likely
adherence to hepatitis C virus treatment
|
|
|
Journal of Viral Hepatitis, Sept 2011
Article first published online: 22 JUN 2010
A. Brett Hauber,1 A. F. Mohamed,1 C. Beam,2 J. Medjedovic3* and J. Mauskopf1 1RTI International, RTP, NC; 2Human Genome Sciences, Inc., Rockville, MD, USA; and 3Novartis Pharma AG, Basel, Switzerland
Abstract
Summary. To estimate patient preferences for attributes of hepatitis C virus (HCV) treatment and patients' assessment of the likely effect of treatment attributes on treatment adherence, HCV patients ≥18 years old completed an online survey that included nine 2-alternative choice questions. Each choice question was defined by the probability of sustained viral response (Efficacy), injection frequency (Frequency), duration of flu-like symptoms after every injection (Flu), injection device (Device), average number of days of work missed each week (Lost Work Days), probability of reversible hair thinning while on treatment (Alopecia) and probability of developing clinical depression while on treatment (Depression). We estimated a mean relative importance weight for each attribute. Patients also answered three rating questions to assess the extent to which treatment attributes might affect adherence. Hundred and fifty patients completed the survey. Efficacy was the most important attribute with a mean relative importance weight of 10 [95% CI: 7.9-12.1]. The remaining attributes were ranked in order of importance as follows: Depression (4.4 [95% CI: 3.6-5.1]), Flu Days (Frequency x Flu) (3.7 [95% CI: 2.2-5.3]), Lost Work Days (2.9 [95% CI: 2.3-3.5]), Alopecia (1.3 [95% CI: 0.7-1.9]) and Device (1.2 [95% CI: 0.4-2.0]). Patients with prior treatment experience were less likely to indicate that treatment attributes would affect adherence. Patients also indicated that increases in the number of flu days would increase the likelihood of nonadherence to treatment. Sustained viral response is the most important treatment attribute to patients but treatment side effects might affect treatment adherence.
Abbreviations:
DCE discrete choice experiments
GED general equivalency diploma
HCV hepatitis C virus
Introduction
Chronic infection with the hepatitis C virus (HCV) is a common sequelae to acute HCV infection with a prevalence of 1.4% in the United States [1,2]. Currently, treatment of patients with chronic HCV infection involves the use of drugs that stimulate the immune system and drugs with antiviral activity. The unmodified interferons (alpha and beta) and pegylated interferon (alpha) are primarily immunomodulators, are given through subcutaneous injection and have side effects such as flu-like symptoms and depression. The only currently used antiviral drug, ribavirin, has a synergistic antiviral effect when combined with peginterferon [3]; it is taken orally and has side effects that can be upsetting (rash/itching) or medically serious (anaemia).
A recent review of the studies of adherence to treatment for chronic HCV infection identified five studies that estimated patient adherence to drug treatment with pegylated interferon and ribavirin combination therapy [4]. These studies indicated that adherence was generally high (70-98%) initially, was higher for pegylated interferon than for ribavirin and decreased over time for both drugs. For example in Smith et al. [5], the percentage of people who took all doses of peginterferon in the prior 4 weeks, measured using electronic monitoring devices, declined from 92% at 4 weeks to 74% at 48 weeks. In the same study, patient self-reported adherence to both drugs was higher than that recorded by the electronic monitoring device.
Results describing the relationship between treatment adherence and sustained viral response are mixed. McHutchison et al. [6] showed that among patients treated with peginterferon plus ribavirin, 63% of those with HCV genotype 1 and adherence >80% achieved sustained viral response, while only 34% of those with the same genotype, but adherence rates ≤80%, achieved the same outcome. Lo Re et al. [7] showed a lower rate of HCV suppression at 12 weeks for those with adherence of <85%. Cacoub et al. [8] showed a trend toward a higher rate of sustained viral response among patients completing a patient-education programme. In contrast, a study by Weiss et al. [9] showed no difference in sustained viral response with different levels of adherence.
The increasing rate of nonadherence over the treatment time period suggests that viral response may not be the only important attribute of treatment to patients with chronic HCV. Specifically, nonadherence may indicate that patients may be willing to risk reducing the probability of achieving sustained viral response to avoid treatment side effects. To test this hypothesis, we developed a choice-format conjoint analysis survey instrument to elicit the willingness of patients to trade-off among different attributes of chronic HCV infection drug treatment. We also elicited patients' assessments of the extent to which treatment attributes might affect adherence.
Methods
Survey instrument
Choice-format conjoint analysis methods - also known as discrete choice experiments (DCE) - have been widely used and validated in transportation research, marketing research and environmental economics. Over the past decade, choice-format conjoint analysis has been used increasingly to quantify preferences for features of health, health care and health care policy [10]. Choice-format conjoint analysis, as applied to healthcare decision-making, is a systematic method of eliciting trade-offs to quantify the relative importance patients (or physicians or other healthcare decision makers) assign to various treatment attributes or outcomes. It is based on the premise that medical interventions are composed of a set of attributes or outcomes (efficacy, safety, mode of administration, etc.) and the attractiveness of a particular intervention to an individual is a function of these attributes [11-16].
Based on a review of the published literature describing the outcomes of current HCV treatments and face-to-face interviews with eight patients with chronic HCV, we identified seven treatment attributes to describe the HCV treatment alternatives in this study: the probability of sustained viral response (Efficacy) ranging between 40% and 70%, injection frequency (Frequency) ranging between once a week to once every 4 weeks, duration of flu-like symptoms after every injection (Flu) ranging between 1 and 3 days, injection device (Device) ranging between a vial and syringe and a pen injection device, average number of days of work missed each week (Lost Work Days) ranging between no days and 2 days, probability of reversible hair thinning while on treatment (Alopecia) ranging between no chance and 40%, and probability of developing clinical depression while on treatment (Depression) ranging between 5% and 25%. In each treatment choice question, patients were asked to choose between two hypothetical HCV treatment profiles in which each profile was defined by varying levels of the seven treatment attributes (Fig. 1). In addition, the choice-format conjoint survey elicited standard demographic information (e.g., age, gender, race, marital status and education) as well as a number of items about the patients' experiences with HCV and HCV treatments.
The survey also included three adherence rating questions to assess how often patients thought they might miss or skip doses of treatments with different attributes (Fig. 2). In each adherence rating question, patients were presented with two HCV treatment profiles and asked to rate the relative likelihood that they would miss or skip doses of the hypothetical treatments. This likelihood was rated on a 5-point Likert scale ranging from 'Much more likely to miss or skip doses with Medicine A' to 'Much more likely to miss or skip doses with Medicine B'. The middle level on the Likert scale was 'Equally likely to miss or skip doses with Medicine A and Medicine B'.
To create treatment profiles for the choice questions, we employed a commonly used algorithm to construct an experimental design resulting in 27 choice pairs [17-21]. The final experimental design consisted of three survey versions or blocks, each containing nine choice pairs. Each subject was randomly assigned to one of the three blocks. The survey was approved by RTI International's Office of Research Protection and Ethics (Research Triangle Park, NC, USA).
Survey sample
All subjects were required to have a self-reported diagnosis of HCV and be a US resident aged 18 or older. All subjects provided informed consent to participate in this study. Synovate HealthCare Research recruited 150 subjects from its existing online chronic illness panel and administered the survey in August 2008. Sample size calculations represent a challenge in conjoint analysis. Minimum sample size depends on a number of criteria, including the question format, the complexity of the choice task, the desired precision of the results and the need to conduct subgroup analyses [22]. Researchers commonly apply a rule of thumb such as that proposed by Orme [23]. A sample size of 150 subjects is consistent with other studies involving surveys of similar complexity [24].
Statistical analysis
We used multivariate, random-parameters or mixed-logit regression to estimate an importance weight for each treatment attribute. In random-parameters logit, the independent variable is treatment choice, and a regression equation is used to estimate the effect of each attribute on the probability of choosing a treatment alternative. Random-parameters logit avoids potential estimation bias from unobserved preference heterogeneity in discrete choice models by estimating a distribution of preferences across patients for each preference parameter [25,26]. In addition, because each subject provided responses to more than one choice question, we estimated a random-effects panel model to account for within-subject correlation. Statistical analyses for the choice models were conducted using NLOGIT 4.0 (Econometric Software, Inc., Plainview, NY, USA).
The relative importance weight for each attribute was estimated over the range of levels of that attribute included in the study. For example, the importance of the Efficacy attribute was estimated as the importance of increasing the probability of achieving sustained viral response from the lowest level (40%) to the highest level (70%). The same approach was used to estimate the relative importance for each attribute with numerical levels. In the case of the Device attribute in which the levels are categorical, the relative importance weight was calculated as the importance of switching from using a vial and syringe to using a pen injection device. The estimated coefficients in a choice model reflect the marginal effect of a one-unit change in an attribute on the probability of choosing a treatment alternative. To facilitate the interpretation of the results, we assigned an importance weight of 10 to the most important attribute and calculated the importance weight for each of the other attributes relative to the importance of the most important attribute.
The adherence rating questions included in the survey asked patients to indicate how much more likely they would be to miss or skip doses of one treatment compared to another. One possible response to this question is 'Equally likely to miss or skip doses with Medicine A and Medicine B'. Choosing this response indicates that either the patient believes that he or she will not miss or skip doses with either treatment or that the attributes of the two treatments do not differ enough to induce a person to be less adherent with one treatment than with the other. In either case, choosing this response provides no information about the extent to which treatment attributes might affect adherence. Therefore, we used a Heckman two-stage model to estimate first the effect of patient characteristics on the likelihood of choosing the response, 'Equally likely to miss or skip doses with Medicine A and Medicine B' and then the effect of treatment attributes on patients' assessment of the likelihood that he or she would miss or skip doses [27-29]. In the first stage, the dependent variable indicated whether a patient chose a response other than 'Equally likely to miss or skip doses with Medicine A and Medicine B' and patient-specific characteristics are used as explanatory variables. In the second stage of the adherence rating model, patients' ratings of the likelihood of missing or skipping doses were modelled as a function of treatment attributes. Specifically, we estimated an ordered-probit model in which the levels of the treatment attributes were used to predict the likelihood of choosing an adherence rating incorporating the results of the first stage by controlling for those who indicated that treatment attributes would likely not affect treatment adherence. Statistical analyses for the adherence models were conducted using STATA 8.2 (Statacorp, College Station, TX, USA).
Results
Survey sample
Synovate Healthcare Research recruited 150 patients, all of whom consented to participate in the research study. The gender of the full sample was split evenly between males and females. The majority of patients (86%) were white, and approximately 45% of patients were married. The mean age was 48 (SD = 9.8). Of the 150 patients, 10% were currently on their first course of therapy (receiving treatment for the first time), 47% were treatment-naïve (never received treatment) and 43% were treatment experienced (currently on treatment but not for the first time, or received treatment in the past). The majority of treatment-experienced patients took injections once a week (53%), experienced flu-like symptoms 1-3 days after every injection (75%), worked full time (56%), had alopecia (60%) and felt depressed (68%) while on their last course of treatment. Table 1 presents some summary statistics on the survey sample.
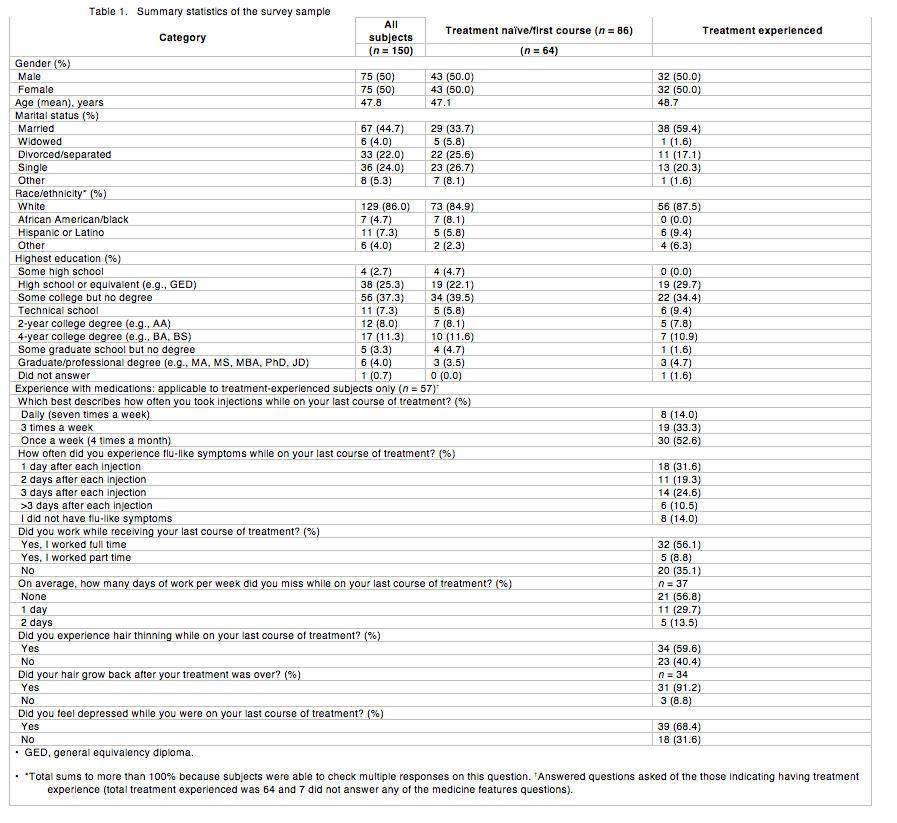
All 150 patients answered nine choice questions. Of the 150 patients, seven patients (5%) had no variation in their responses. That is, they chose the same response (Medicine A or Medicine B) in all nine choice questions. The lack of variation in the responses may indicate that these patients were not paying attention to the choice task. These seven patients were deleted from the final data set used to estimate the choice and adherence models.
Mean relative importance weights
Preliminary analyses of the data indicated that the impact of flu-like symptoms on treatment choice is best expressed as the number of flu days (Flu Days) every month [defined as the product of injection frequency and the duration of flu-like symptoms after every injection (Frequency x Flu)]. Specifically, when Flu, Frequency and Flu Days were all included in the model, only Flu Days was statistically significant.
Figure 3 presents the mean relative importance weight (and 95% confidence interval) for each treatment attribute. Over the range of levels of each attribute in the study, Efficacy was the most important attribute with an importance weight of 10 [95% CI: 7.9-12.1]. The remaining attributes were ranked in order of importance as follows: Depression (4.4 [95% CI: 3.6-5.1]), Flu Days (Frequency x Flu) (3.7 [95% CI: 2.2-5.3]), Lost Work Days (2.9 [95% CI: 2.3-3.5]), Alopecia (1.3 [95% CI: 0.7-1.9]) and Device (1.2 [95% CI: 0.4-2.0]). While mean relative importance differed among all attributes, many of these differences were not statistically significant. Efficacy was statistically significantly more important than the other five attributes (P < 0.05). Depression, Flu Days and Lost Work Days were statistically significantly more important than Alopecia and Device (P < 0.05). Importance weights for Depression, Flu Days and Lost Work Days were not statistically significantly different from each other at the 5% level of significance.
These results indicate that a 30%-point improvement in the probability of sustained viral suppression (Efficacy) (from 40% to 70%) was, on average, more than twice as important as decreasing the probability of clinical depression by 20% points (from 25% to 5%) or reducing the number of days with flu-like symptoms from 12 per month (four injections per month times 3 days of flu-like symptoms after each injection) to 1 per month (one injection per month with 1 day of flu-like symptoms after the injection). In addition, decreasing the number of Flu Days from 12 per month to 1 per month was, on average, more than three times as important as switching from vial and syringe to an injection device or eliminating the possibility of hair thinning (Alopecia). Finally, reducing the probability of clinical depression from 25% to 5% was approximately 1.5 times as important as reducing the number of days missed from work from 2 days per week to 0 days per week.
Adherence rating results
The first stage of the adherence rating model indicated that prior treatment experience was the only patient-specific characteristic that influenced the likelihood that a patient will choose something other than 'Equally likely to miss or skip doses with Medicine A and Medicine B'. Specifically, patients with prior treatment experience were less likely to state that treatment attributes will affect the likelihood of missing or skipping doses than patients without prior treatment experience. In the second stage of the model, the only treatment feature to increase the likelihood of missing or skipping doses was increases in the number of flu days (either from increases in the number of injections or increases in the number of days of flu-like symptoms after every injection)
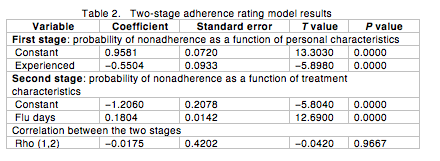
Table 3 presents the likely effect of Flu Days on patients' ratings of the likelihood of missing or skipping doses, controlling for the fact that some patients indicated that treatment attributes likely would not affect treatment adherence. As the likelihood of Flu Days increases, likely adherence decreases. Predicted nonadherence increased as the number of Flu Days increases, but at a decreasing rate. That is, each additional flu day had a smaller effect on adherence ratings than the previous flu day. These results can be interpreted as the relative effect of Flu Days on adherence. That is, assuming adherence to treatments with no flu-like symptoms is less than perfect, these results demonstrate the effect of increasing Flu Days on adherence. For example, if adherence to treatments with no flu-like symptoms is 80% (baseline adherence rate = 80%), adding 4 days of flu-like symptoms decreases predicted adherence by 13.6% to yield an overall adherence rate of 66.4% (80% x 83%).
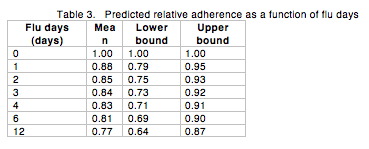
Discussion
The results of this study indicate that sustained viral response was the most important attribute of a drug treatment to patients. However, the results of the choice-format conjoint survey also indicate that patients were willing to accept decreases in the probability of achieving sustained viral response to reduce side effects of treatment. Alopecia was the least important of the side effects included in this analysis, while depression and days with flu-like symptoms were relatively more important to patients. The extent to which treatment interferes with work was also an important consideration for patients. In addition, both patient characteristics (prior treatment experience) and treatment attributes (the number of days with flu-like symptoms) affected patients' ratings of likely adherence.
Using adaptive conjoint analysis, Fraenkel et al. [30] also found that treatment benefit (measured as virus eradication in 45% of patients with no cirrhosis after 10 or more years) was the most important HCV treatment attribute among treatment-naïve patients, whereas fatigue, depression and flu-like illness had similar but smaller influences on subjects' preferences. However, patients with moderate or severe fibrosis placed a greater importance on treatment benefit and less on treatment-related side effects than patients with mild or no fibrosis. Significant differences between our study and Fraenkel et al. [30] are that we included different treatment attributes and both treatment-naïve and treatment-experienced patients.
There have been several nonconjoint studies published on HCV patients' preferences [31-34]. Two studies looked at patients' role in decision-making regarding initiating treatment [31,32]. Fraenkel [31] concluded that physicians need to determine patients' levels of uncertainty in decision-making, whereas Tine [32] reported that chronic HCV patients defer to their physicians in initiating treatment. Schackman et al. [33] found that treatment-naïve patients were more concerned about side effects than physicians and nurses using standard gamble. Witkos et al. [34] examined claims data of Canadian HCV patients and concluded that disease severity, age, HIV status and province of residence increased the likelihood of receiving treatment.
One of the strength of this study is in the use of a choice-format conjoint survey that reflects the attributes of current drug treatments for chronic HCV infection based on published literature and eight face-to-face subject pretest interviews. The results from well-designed choice-format conjoint analyses in health economics have been shown to provide useful information about the relative importance of different treatment attributes to patients and physicians [24,35,36]. Another strength of the study is the use of an innovative method to estimate the likely impact of different medication attributes on adherence. This method provides quantitative estimates that can be used to predict adherence to new medications that have different attributes from current medications [37,38]. Although the impact of patient characteristics and treatment attributes on patients' adherence ratings was estimated in our study using hypothetical scenarios, the results of this study are consistent with previously published results that indicate that nonadherence is owing to treatment-related adverse events for 75% of patients with poor adherence [6]. Because our estimation method gave results that were consistent with observed determinants of adherence to treatment for chronic HCV infection, these estimates could be used in economic models of the cost-effectiveness of a new treatment regimen compared to current treatments. Adherence could be included in these models in an attempt to convert the efficacy results seen in clinical trials to effectiveness in 'real-world' practice.
While choice-format conjoint analysis methods are widely used in health economics to elicit preferences for treatment features and outcomes, they have limitations. One inherent limitation is that subjects evaluate hypothetical treatments. These constructed choice questions are intended to simulate possible clinical decisions but do not have the same clinical, financial and emotional consequences of actual decisions. Thus, differences can arise between stated and actual choices. We have attempted to minimize such potential differences by offering alternatives that mimic real-world trade-offs as closely as possible. In addition, some health professionals are skeptical that people have sufficient understanding of treatment information to competently evaluate treatment alternatives. Diagnosis among subjects in this study was self-reported and not confirmed by physician consultation or chart review. However, we believe the likelihood is small that people without HCV would be likely to complete a study such as this because the study is cognitively challenging and requires an investment in time in exchange for little personal gain. Finally, we recruited subjects through an Internet-based chronic illness panel which means that the sample used in this study is not necessarily representative of the people with HCV in the United States.
Because the treatment alternatives that patients considered and the patients' adherence ratings are based on hypothetical treatment scenarios, the adherence results presented in this study should not be construed as an absolute prediction of the rate of nonadherence to treatment. First, there likely is some underlying rate of nonadherence that is not explained by patient characteristics (prior treatment experience) or treatment attributes (days of flu-like symptoms). Therefore, our estimate of predicted adherence to treatment based on these adherence ratings must, at a minimum, be interpreted as the relative effect of known patient or treatment characteristics on adherence. In addition, a previous study found that self-reported adherence was greater than measured adherence in this patient population indicating that patients underestimated their own rate of nonadherence or were unwilling to acknowledge the level of their nonadherence [5]. If this is the case, the adherence estimates from this study may represent a lower bound on the impact of the number of days of flu-like symptoms on nonadherence. In summary, the results of our analyses have shown that, although efficacy is clearly the most important treatment attribute, other attributes related to treatment side effects are also important and may reduce adherence to current treatments. Because adherence to therapy, especially in those with genotype 1 HCV infections, has been shown to be important for achieving a sustained viral response, new treatment regimens that reduce side effects relative to current treatments might result in higher adherence rates and better clinical effectiveness.
|
|
|
|
|
|
|